Large Language Models (LLMs), like GPT-4, LLaMA, BERT, and ChatGPT, have garnered significant attention and interest in recent years due to their remarkable capabilities in natural language processing (NLP) and generation. As these models continue to evolve and find applications in various domains, it’s important to separate fact from fiction when it comes to their abilities and limitations. In this article, we will delve into and debunk seven common myths surrounding LLMs.
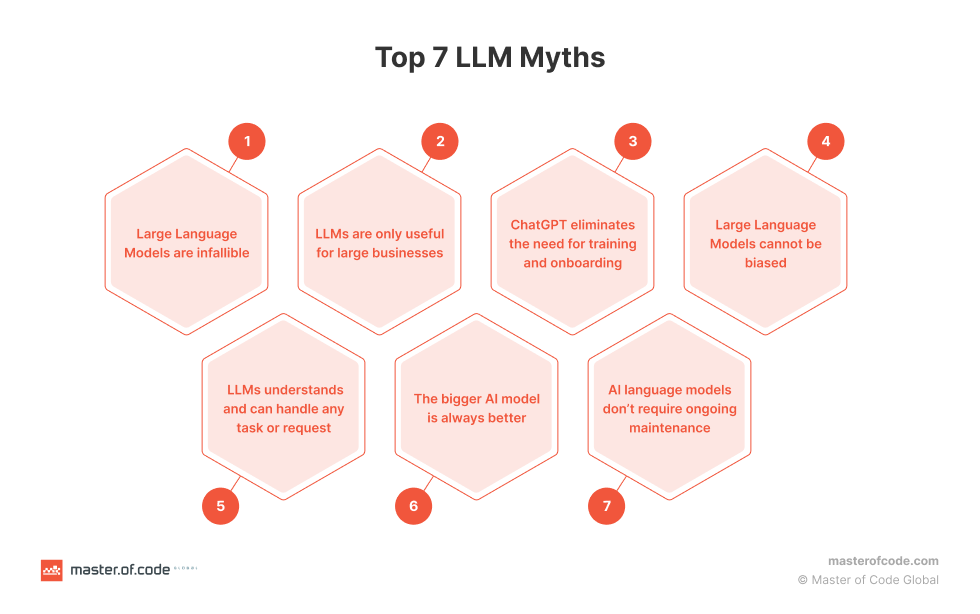
Table of Contents
LLMs Myth 1: Large Language Models are infallible
While LLMs have demonstrated remarkable capabilities in generating human-like text, it is crucial to dispel the myth that they are infallible. LLMs occasionally produce incorrect or nonsensical outputs, commonly known as “hallucinations,” which can have detrimental consequences for companies’ reputation and the spread of inaccurate information online.
The hallucination rate for ChatGPT, one of the widely known LLMs, has been estimated to be around 15% to 20% This indicates that a significant portion of the generated text may be erroneous or detached from reality. The consequences of LLM hallucination are significant, as inaccuracies in generated text can contribute to the spread of misinformation and disinformation. A survey conducted by Telus revealed that 61% of respondents expressed concerns about the increased dissemination of inaccurate information online. An alarming example of LLM hallucination occurred when a New York lawyer included fabricated cases generated by ChatGPT in a legal brief filed in federal court, highlighting the potential risks associated with relying solely on LLM-generated information.
Various factors contribute to the occurrence of hallucinations in LLMs, including source-reference divergence, exploitation through jailbreak prompts, reliance on incomplete or contradictory datasets, overfitting, lack of novelty, and guesswork from vague or insufficiently detailed prompts. According to some studies, LLM hallucinations often manifest in self-contradictions, where the generated text presents conflicting answers, eroding consistency and trustworthiness. ChatGPT, for example, exhibits a self-contradiction rate of 14.3%, while GPT-4 demonstrates a rate of 11.8%. Factual contradictions further emphasize the fallibility of LLMs. Research on hallucinations in ChatGPT indicates that ChatGPT 3.5 had an overall hallucination rate of approximately 39%, while ChatGPT 4 exhibited a hallucination rate of approximately 28%. These statistics emphasize the occurrence of incorrect or misleading responses generated by these versions of ChatGPT, which is considered one of the main Generative AI and LLMs’ challenges.
Want to mitigate the challenges of hallucination in LLMs and enhance your chatbot? Discover LOFT (LLM Orchestration Framework Toolkit) by Master of Code. Our innovative solution empowers you to mitigate hallucination in LLMs, raise chatbot efficiency, and drive impactful, engaging experiences for your customers. Seamlessly integrate LOFT into your existing framework to elevate your chatbot’s performance and revolutionize user experiences.
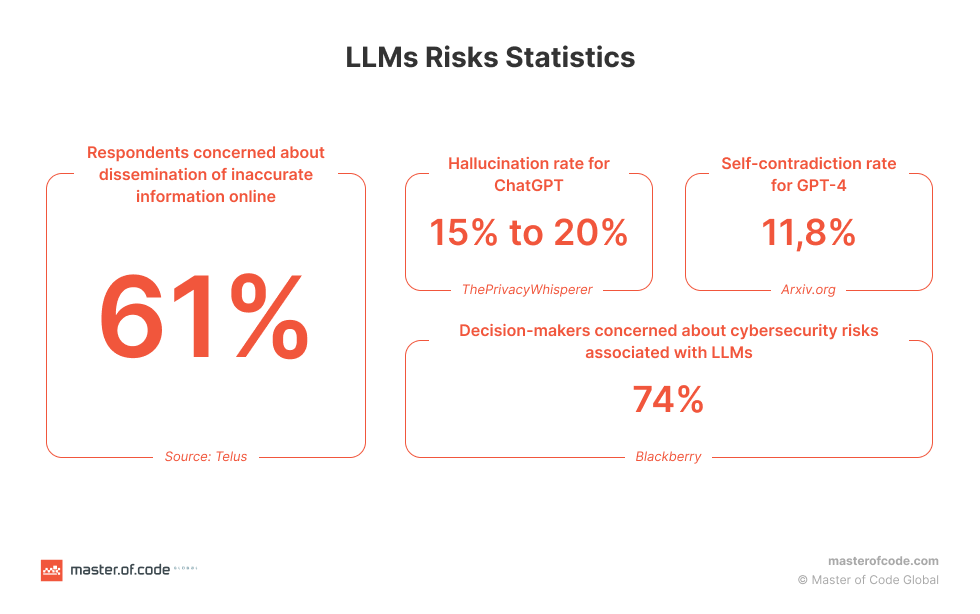
The consequences of LLM hallucination extend beyond inaccuracies. Privacy issues arise as LLMs may generate toxic or discriminatory content, one of the greatest red flags in telecom, banking, and finance industries. For instance, ChatGPT’s persona assignment has been observed to perpetuate harmful stereotypes, leading to a six-fold increase in toxicity. Moreover, concerns about cybersecurity risks associated with LLMs, including the potential for spreading misinformation, have been voiced by 74% of IT decision-makers who are interested in solutions with LLMs.
The evidence presented clearly debunks the myth that large language models are infallible. The occurrence of hallucinations in LLMs underscores the need for caution and critical evaluation when relying on their outputs. It is crucial to recognize the limitations of these models and approach their results cautiously. By enhancing their reliability and mitigating the consequences of inaccuracies and misinformation, we can realize the full potential of LLMs while ensuring their informed and responsible usage.
LLMs Myth 2: LLMs are only useful for large businesses
One of the most common misconceptions about LLMs is that they are only useful for large businesses. In fact, LLMs can be a valuable tool for small and midsize businesses as well. For example, according to a survey conducted by GoDaddy with 1,003 small business owners in the United States, 27% of respondents reported using Generative AI tools. Notably, ChatGPT emerged as the most commonly utilized tool for business purposes, with 70% of small business owners leveraging its capabilities to improve efficiency, enhance decision-making, get more personalized and engaging experiences for users, and reduce spendings.
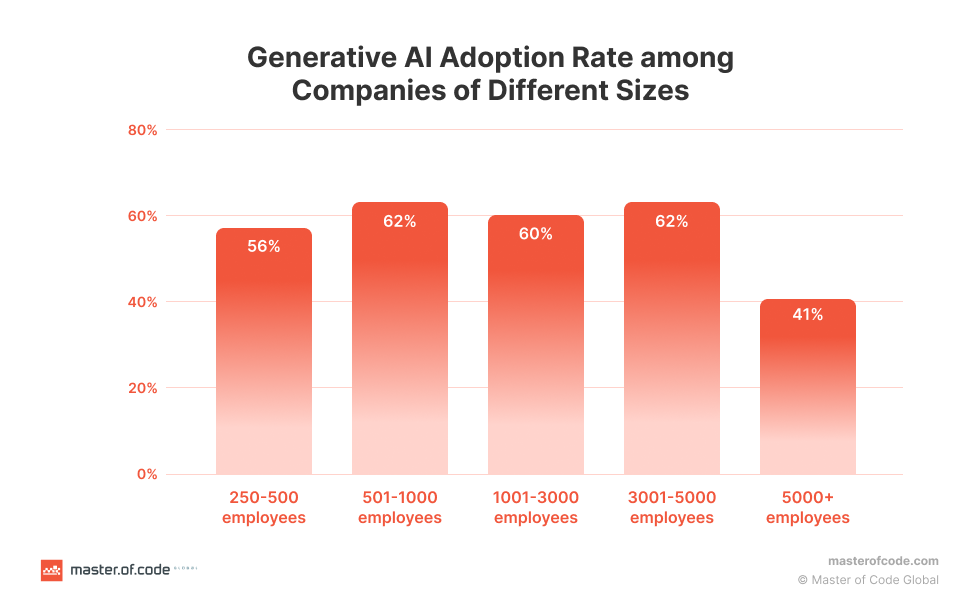
Furthermore, data from a survey by Upwork reveals that midsized companies are leading the adoption of Generative AI. Among companies with 501-5,000 employees, an impressive 62% reported utilizing Generative AI. Comparatively, 56% of small companies (251-500 employees) and 41% of larger companies (5,001+ employees) indicated the adoption of Generative AI tools, like ChatGPT. These findings indicate that the use of LLMs and Generative AI is not exclusive to large businesses, with mid-sized and small companies actively using these technologies to maximum advantage.
LLMs Myth 3: ChatGPT eliminates the need for training and onboarding
The claim that ChatGPT eliminates the need for training and onboarding is a dangerous misconception. While ChatGPT can be a valuable tool for training and onboarding, it is not a replacement for these essential processes. ChatGPT or any other LLM is not a perfect replacement for training and onboarding because it is a machine learning (ML) model, it cannot provide personalized attention, and it cannot replace training materials.
Moreover, employing Generative AI models, like ChatGPT, introduces risks such as data exposure and breaches. Approximately 11% of the information shared with ChatGPT by employees consists of sensitive data, posing potential threats to data security and privacy. This underscores the importance of providing proper training and guidelines to employees to mitigate these risks. Furthermore, a study conducted by Epignosis revealed that 35% of employees in the United States reported changes in their work responsibilities due to AI tools. Consequently, 49% of employees expressed the need for training on using AI tools like ChatGPT, yet only 14% reported receiving such training. These findings highlight the necessity for organizations to provide comprehensive training and onboarding programs to equip employees with the skills and knowledge required to effectively utilize AI tools like ChatGPT.
Fortunately, organizations are increasingly recognizing the need for guidelines and policies to
ensure ethical and legal use of Generative AI tools. In fact, 68% of companies are establishing guidelines and criteria for responsible Generative AI within organizations. This includes training employees on permitted data usage, safe practices, and validating outputs to eliminate biases. Additionally, organizations are establishing sustainability guidelines to determine when Generative AI is the best solution and providing employees with decision frameworks or checklists to evaluate its implementation.
LLMs Myth 4: Large Language Models are immune to biases
Biases in LLMs can be attributed to the training process and the data sources used. LLMs, including ChatGPT, rely on unsupervised learning and learn patterns from vast amounts of unlabelled data sourced from the internet. This data consists of diverse text corpora, including websites, articles, books, and other written content. As noted by researchers from OpenAI describing the training of an earlier LLM, GPT-3, the training mix consisted of 60% internet-crawled material, 22% curated content from the internet, 16% from books, and 3% from Wikipedia. While ChatGPT is based on updated models (GPT-3.5 and GPT-4) where the specific percentages may differ, it is evident that some training data originates from biased sources.
The data used in ChatGPT undergoes preprocessing and filtering to remove low-quality content, explicit material, web and social spam, and other undesired text. However, due to the vast scale of the data and the limitations of current filtering techniques, some biases may still penetrate the training dataset. This can give rise to various types of biases in the training data, including demographic, cultural, linguistic, temporal, ideological, and political biases. These biases, such as over- or under-representation of certain demographic groups, presence of cultural stereotypes, limited support for minority languages, lack of understanding of historical contexts, and absorption of political biases, can infiltrate LLMs during the learning process.
When adopting the new technology, it is important to acknowledge that biases can persist and influence the outputs generated by LLMs, despite efforts to mitigate biases through preprocessing and filtering. The limitations in filtering techniques and the presence of biases in the training data highlight the need for ongoing research, improved filtering methods, and ethical considerations to address and mitigate biases in LLMs, ensuring the development of more fair and unbiased language models.
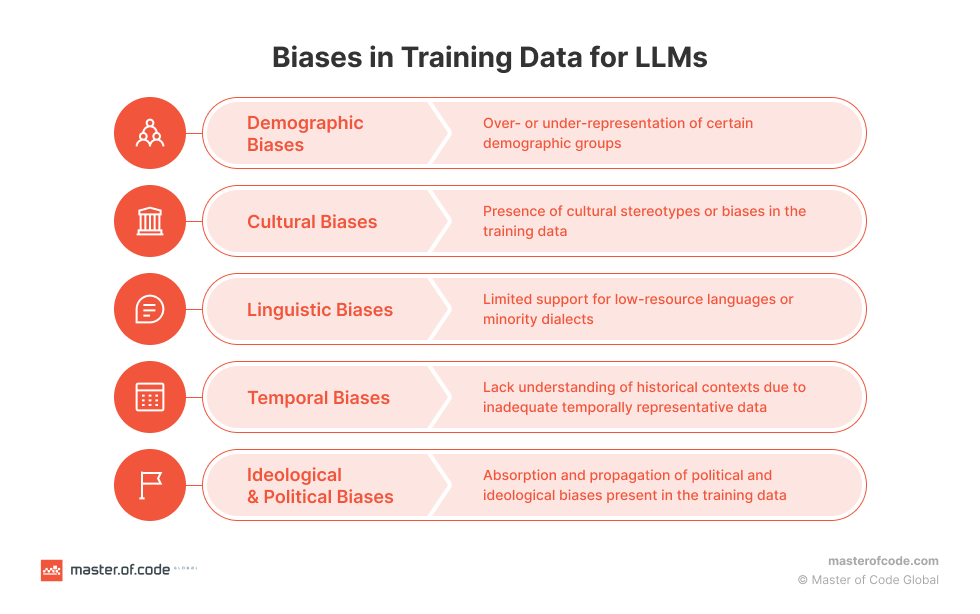
LLMs Myth 5: LLMs understands and can handle any task or request
While LLMs like ChatGPT have demonstrated impressive language capabilities, it is important to dispel the myth that they can understand and handle any task or request. There are several limitations to consider when relying on LLMs for various use cases:
- Business-specific use cases: LLMs like ChatGPT cannot effectively solve business-specific use cases without undergoing substantial training. While they possess general language understanding, they lack domain-specific knowledge and expertise required for complex business tasks. However, through the strategic application of prompt engineering techniques, businesses can provide targeted instructions and context to guide the LLM’s generation process.
- Limited knowledge and nuances: LLMs may struggle with tasks that involve common sense knowledge, legal or professional advice, or understanding nuanced language elements such as sarcasm or humor. Their training data primarily consists of internet text, which may not cover specialized knowledge domains or capture the full breadth of human communication.
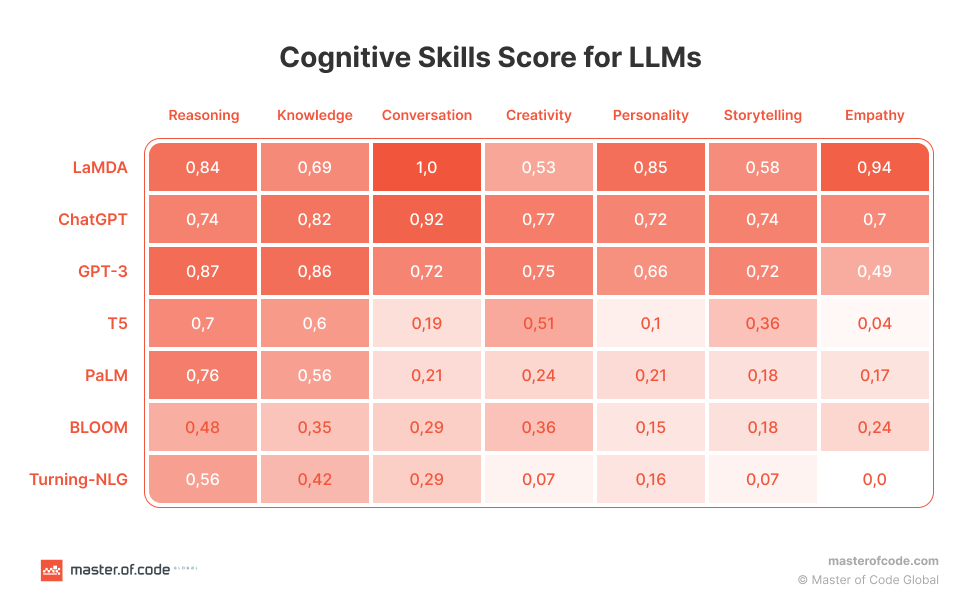
- Temporal Constraints: LLMs like ChatGPT have a cutoff point in their training data, typically up until a specific year (e.g., 2021). Consequently, they may not possess up-to-date information on recent events, advancements, or changes that have occurred beyond their training period.
- Dependency on Web Lookup: LLMs are not equipped to perform real-time web searches or provide information beyond their pre-existing training data. They rely solely on the information available during training and cannot access the internet in real-time to retrieve new or updated data.
- Accuracy and Reliability: While LLMs strive to generate accurate responses, they are not infallible. There is always a possibility of errors, misconceptions, or incorrect outputs. It is essential to exercise caution and verify information obtained from LLMs to ensure its accuracy and reliability.
It is important to recognize that LLMs have their limitations and should be utilized within their appropriate scope, for example LLMs can be suitable for development of Generative AI chatbots. While they excel at certain language-related tasks, they may not possess the comprehensive understanding or capabilities to handle every task or request. Careful consideration and context-specific solutions are necessary to maximize their benefits while addressing their limitations.
LLMs Myth 6: The bigger AI model is always better
Contrary to popular belief, the notion that a bigger AI model is always superior is misleading. While the size of a model is often associated with its performance, other factors come into play that can significantly impact its effectiveness:
- Training Data Quality: Research suggests that there can be differences in the generation of inaccurate information between larger and smaller models. For example, studies have shown that the 6B-parameter GPT-J model was found to be 17% less accurate than its 125M-parameter counterpart, indicating potential challenges associated with larger models. This highlights the importance of not solely relying on the size of the model for improved performance. By carefully preparing and curating the training data, it is possible to improve the model’s quality while reducing its size. One example is the T0 model, which is 16 times smaller than GPT-3 but outperforms it on various benchmark tasks. This showcases that focusing on the quality and relevance of the training data can have a more significant impact on model performance than simply increasing its size.
- Task-Specific Optimization: Each AI model is designed for specific use cases, like use cases for marketing or use cases in call centers where it can provide maximum benefits. For example, LaMda or GPT-3 are best suitable for Conversational AI, whereas BLOOM works best for machine-translation and content generation. Fine-tuning the model to adapt to specific tasks and contexts is crucial for achieving optimal performance. It is not solely the size of the model that determines its effectiveness but rather its ability to address the specific requirements and nuances of the given task.
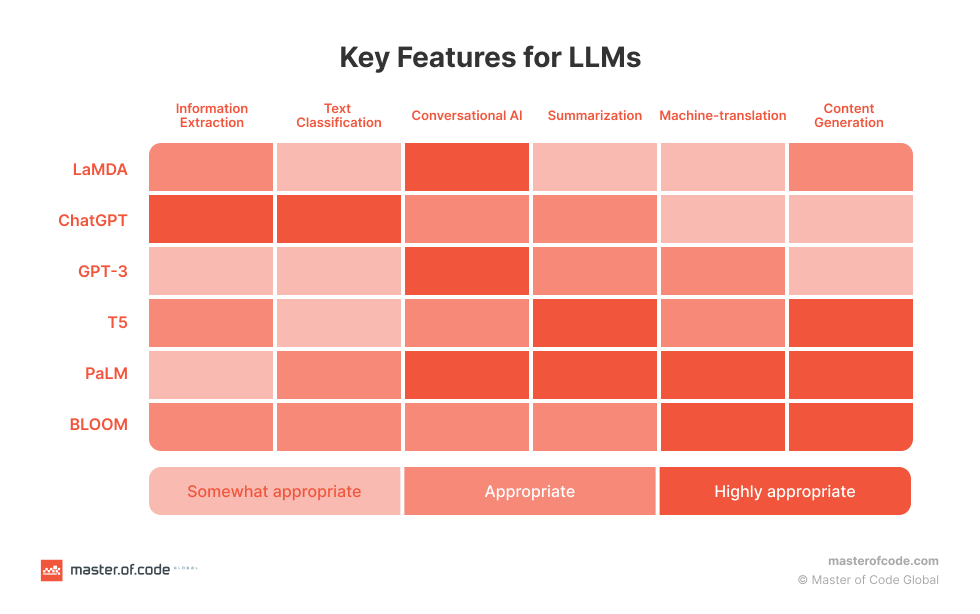
- Risk of Overfitting: Dumping an enormous amount of data into a machine learning model carries the risk of overfitting. When a model is trained with excessive data, it can end up memorizing the information rather than learning the underlying patterns. This can lead to high error rates when the model encounters unseen data. It is essential to strike a balance by providing high-quality, relevant data to ensure the development of the best machine learning model.
In summary, the size of an AI model is not the sole determinant of its performance. The quality and relevance of the training data, the task-specific optimization, and the avoidance of overfitting are all crucial considerations in achieving the desired outcomes. Rather than assuming that a bigger model is always better, it is important to assess the specific requirements of the task and carefully tailor the model’s training and architecture to meet those needs effectively.
LLMs Myth 7: AI language models do not require ongoing maintenance
The utilization of generative artificial intelligence (AI), including LLMs like ChatGPT, has become increasingly popular among businesses. According to recent statistics from IBM, 50% of CEOs are integrating Generative AI, which includes LLMs, into their products and services. However, this widespread adoption is not without its challenges.
One of the main issues associated with LLM adoption is the need for ongoing maintenance. These language models require comprehensive quality checks and human oversight to overcome limitations such as bias and inaccurate information. Regular updates to the training data and fine-tuning of the models are necessary to ensure they continue to provide reliable and accurate outputs. On average, fine-tuning LLMs increases their accuracy by around 10%, highlighting the impact of ongoing maintenance activities. This process involves training the model with domain-specific or task-specific data to optimize its performance for specific use cases, for example, airport chatbot. Monitoring LLM models in production is critical to ensure their ongoing performance and to meet the needs of users. This is where LLMOps (Large Language Model Ops) comes into play. LLMOps, a sub-category of MLOps (Machine Learning Operations), focuses on the operational capabilities and infrastructure required to fine-tune and deploy LLMs effectively.
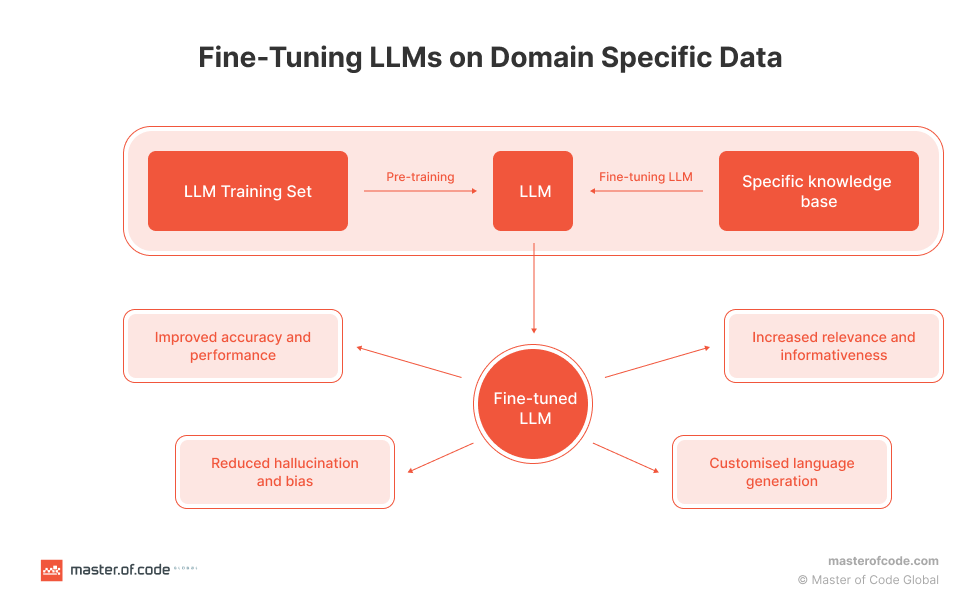
LLMOps enables real-time monitoring of LLMs, providing capabilities such as performance monitoring, anomaly detection, and root cause analysis. With real-time monitoring, organizations can track metrics like accuracy, precision, recall, CPU usage, and memory usage. This helps in identifying any drops in model performance and addressing them promptly. Additionally, LLMOps facilitates governance and compliance, ensuring that LLM models comply with laws and regulations. It provides the necessary infrastructure for ongoing maintenance activities, including data updates, model refinement, and seamless deployment of updated models as part of products and services.
The Bottom Line
When it comes to LLMs, numerous myths surround their capabilities and implications. Some commonly held beliefs include the notion that LLMs are always accurate and unbiased, that only large businesses benefit from them, and that their usage requires minimal maintenance. However, the reality is quite different. LLMs can be prone to inaccuracy and biases due to their internal processes and the biases present in the vast amounts of pre-training data they rely on. Contrary to the myth, businesses of all sizes can leverage LLMs and Generative AI to gain a competitive advantage and various benefits. However, it is crucial for organizations to develop guidelines for the proper use of AI technologies among employees, ensuring responsible and ethical practices.
LLMs are not perfect and may not be able to handle all tasks or requests. However, they excel in specific fields or tasks where they demonstrate exceptional performance. For example, LaMDA, GPT-3, and PaLM are highly suitable for Generative AI chatbots. Moreover, with the right tools and techniques, organizations can maximize the benefits of LLMs in AI chatbots.
For example, Master of Code’s LOFT (LLM Orchestration Framework Toolkit) is a resource-saving solution that enables organizations to integrate Generative AI capabilities into existing chatbot projects without extensive modifications. LOFT seamlessly embeds into the NLU provider and the model, giving organizations greater control over the flow and answers generated by the LLM. LOFT also provides users with the necessary data through external APIs, allowing them to add rich and structured content alongside the conversational text specific to the channel. By using LOFT, organizations can ensure that their chatbots are providing accurate and reliable information, while also being engaging and informative.
Don’t miss out on the opportunity to see how Generative AI chatbots can revolutionize your customer support and boost your company’s efficiency.