The rapid advancements in large language models (LLMs) have revolutionized the field of artificial intelligence, enabling powerful models with the ability to generate text in diverse domains and improve customer experience. However, as LLMs such as ChatGPT continue to evolve, a significant concern arises: hallucination. Hallucination in LLMs refers to the generation of inaccurate, nonsensical, or detached text, posing potential risks and challenges for organizations utilizing these models. The prevalence of hallucination in LLMs, estimated at a rate of 15% to 20% for ChatGPT, can have profound implications for companies’ reputation and the reliability of AI systems. In this article, we delve into the complex nature of hallucination, exploring its definition, underlying reasons, different types, potential outcomes, and effective strategies to mitigate this phenomenon.
Table of Contents
Understanding LLM Hallucination: Definition and Implications
In recent years, the rapid progress of LLMs solutions, exemplified by the remarkable capabilities of ChatGPT, has propelled the field of artificial intelligence into uncharted territory. These cutting-edge models have unlocked the potential for generating accurate text across diverse domains within the given context, revolutionizing the way we interact with AI-powered systems. These advancements have gained significant traction, with 50% of CEOs integrating Generative AI into products and services, as reported by IBM. However, alongside these advancements, a pressing issue that plagues LLMs is known as hallucination. Hallucination in the context of LLMs refers to the generation of text that is erroneous, nonsensical, or detached from reality. The severity of this issue is underscored by a survey conducted by Telus, where 61% of respondents expressed concerns about the increased spread of inaccurate information online. These concerns highlight the urgency and importance of addressing hallucination in LLMs to ensure the responsible Generative AI and LLM technologies use.
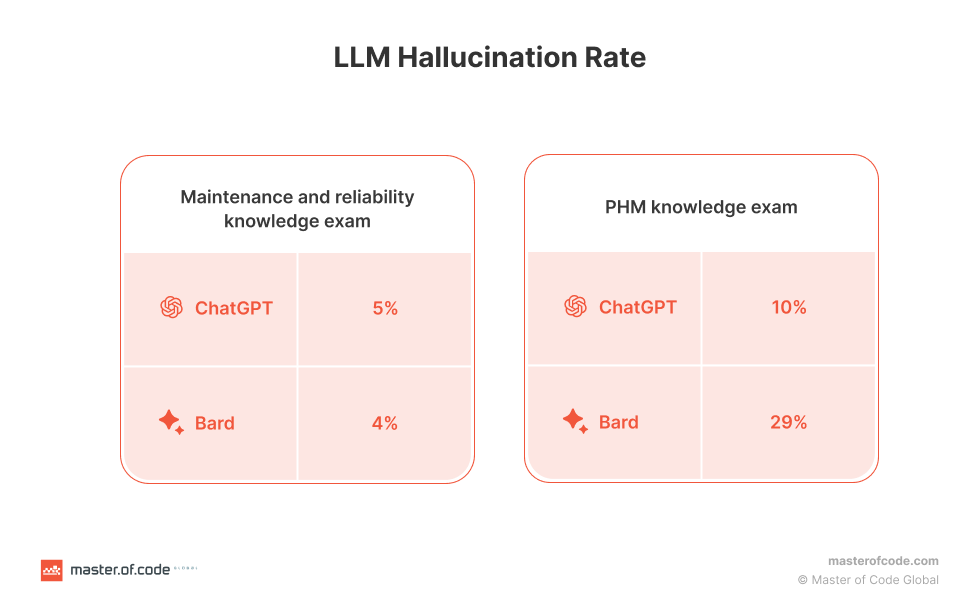
Unlike databases or search engines, LLMs lack the ability to cite their sources accurately, as they generate text through extrapolation from the provided prompt. This extrapolation can result in hallucinated outputs that do not align with the training data. For instance, in a research study focused on the law sector, 30% of trials demonstrated a non-verbatim match with unrelated intent, highlighting the model’s hallucinative tendencies. The limited contextual understanding of LLMs, as they abstract information from the prompt and training data, can contribute to hallucination by potentially overlooking crucial details. Additionally, noise in the training data can introduce skewed statistical patterns, leading the model to respond in unexpected ways.
As AI-driven platforms gain widespread adoption in sectors such as Generative AI in healthcare, Generative AI in banking, and Generative AI in marketing, addressing hallucination becomes increasingly crucial. A notable example of LLM hallucination occurred when a New York lawyer included fabricated cases generated by ChatGPT in a legal brief filed in federal court, emphasizing the potential for inaccuracies in LLM-generated information. Users who rely on these LLMs for critical decision-making or research must be aware of the possibility of false or fabricated information and take necessary precautions to mitigate the associated consequences.
Causes of Hallucination in LLMs
Hallucination in LLMs can be attributed to various factors, each contributing to the generation of false or fabricated information. Delving into the underlying causes of LLM solutions (ex. ChatGPT, Bing, Bard) hallucination provides valuable insights into the factors that contribute to the generation of false or fabricated information, allowing us to better understand their far-reaching implications.
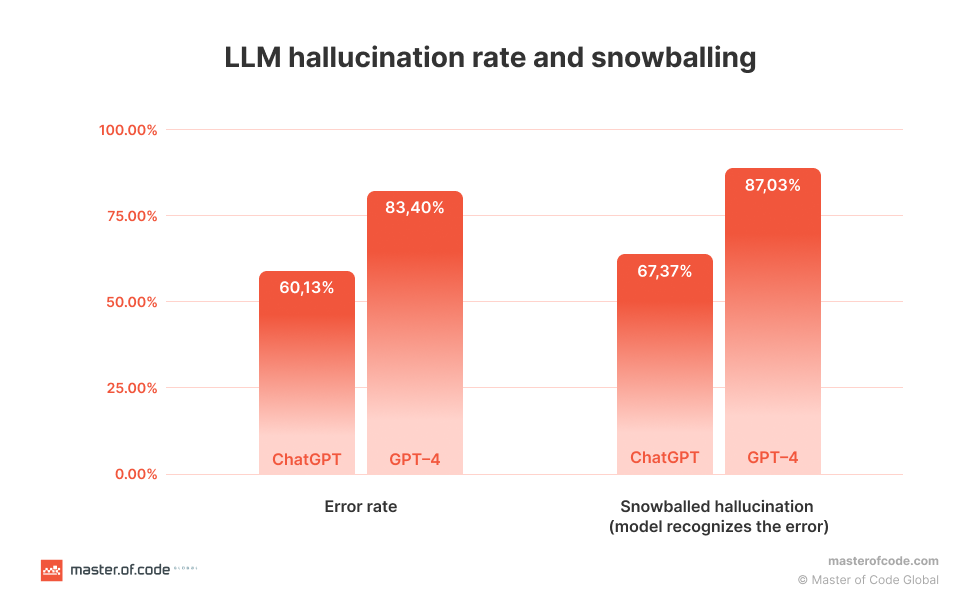
Source-Reference Divergence
One significant cause of hallucination arises from source-reference divergence in the training data. This divergence can occur as a result of heuristic data collection methods or due to the inherent nature of certain NLG tasks. When LLMs are trained on data with source-reference divergence, they may generate text that lacks grounding in reality and deviates from the provided source. This phenomenon highlights the challenge of maintaining faithful representation during text generation.
Exploitation through Jailbreak Prompts
Another contributing factor to hallucination lies in the training and modeling choices made in neural models. LLMs can be vulnerable to exploitation through the use of “jailbreak” prompts. By manipulating the model’s behavior or output beyond its intended capabilities, individuals can exploit vulnerabilities in the programming or configuration. Jailbreaking prompts can result in unexpected and unintended outputs, allowing the LLMs to generate text that was not originally anticipated or predicted.
Reliance on Incomplete or Contradictory Datasets
Additionally, the reliance of LLMs on vast and varied datasets introduces the possibility of hallucination. These datasets may contain incomplete, contradictory, or even misinformation, significantly influencing the LLM’s response. LLMs solely rely on their training data and lack access to external, real-world knowledge. Consequently, their outputs may include irrelevant or unasked details, leading to a breakdown in accuracy and coherence.
Want to mitigate the challenges of hallucination in LLMs and enhance your chatbot? Discover LOFT (LLM Orchestration Framework Toolkit) by Master of Code. Our innovative solution empowers you to mitigate hallucination in LLMs, raise chatbot efficiency, and drive impactful, engaging experiences for your customers. Seamlessly integrate LOFT into your existing framework to elevate your chatbot’s performance and revolutionize user experiences.
Overfitting and Lack of Novelty
Overfitting, a common challenge in machine learning, can also contribute to hallucination in LLMs. Overfitting occurs when the models become too closely aligned with their training data, making it difficult to generate original text beyond the patterns it has learned. This limited capacity for novelty can result in hallucinated responses that do not accurately reflect the desired output.
Guesswork from Vague or Insufficiently Detailed Prompts
Furthermore, vague or insufficiently detailed prompts pose a risk for hallucination. LLMs may resort to guesswork based on learned patterns when faced with ambiguous input. This tendency to generate responses without a strong basis in the provided information can lead to the production of fabricated or nonsensical text.
In summary, LLM hallucination arises from a combination of factors, including source-reference divergence in training data, the exploitation of jailbreak prompts, reliance on incomplete or contradictory datasets, overfitting, and the model’s propensity to guess based on patterns rather than factual accuracy. Understanding these causes is essential for mitigating hallucination and ensuring the reliability and trustworthiness of LLM-generated outputs.
Types of Hallucinations in LLMs: Understanding the Spectrum
Hallucinations in LLMs can manifest in various forms, each highlighting the challenges associated with generating accurate and reliable text. By categorizing these hallucinations, we can gain insights into the different ways in which LLMs can deviate from the desired output and use them to overcome the limitations and transform Customer Experience (CX). Let’s explore some common types of hallucinations.
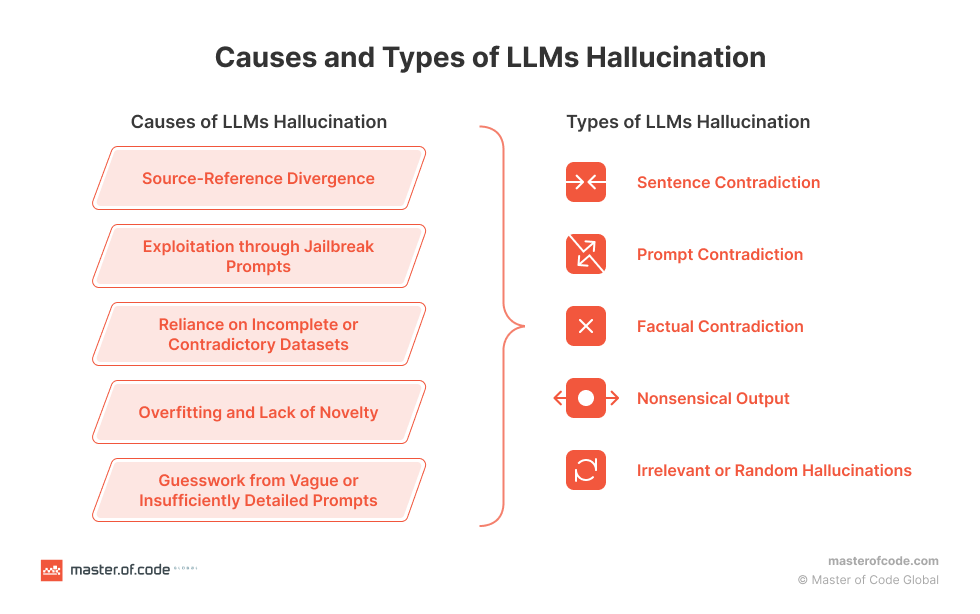
Sentence Contradiction
LLMs have the potential to generate sentences that contradict a previous statement within the generated text, introducing inconsistencies that undermine the overall coherence of the content. These sentence contradictions pose significant challenges in maintaining logical flow and can result in confusion for users relying on the LLM-generated text. Recent research on self-contradictory hallucinations in LLMs indicates a high frequency of self-contradictions, with ChatGPT exhibiting a rate of 14.3% and GPT-4 demonstrating a rate of 11.8%.
Prompt Contradiction
Prompt contradiction occurs when a sentence generated by an LLM contradicts the prompt used to generate it. This type of hallucination raises concerns about the reliability and adherence to the intended meaning or context. Prompt contradictions can undermine the trustworthiness of LLM outputs, as they deviate from the desired alignment with the provided input.
Factual Contradiction
LLMs possess the capacity to generate fictional information, falsely presenting it as factual. Factual contradictions manifest when LLMs produce inaccurate statements that are erroneously presented as reliable information. These hallucinations have profound implications, as they contribute to the dissemination of misinformation and erode the credibility of LLM-generated content.
For instance, a study on hallucinations in ChatGPT revealed that ChatGPT 3.5 exhibited an overall success rate of approximately 61%, correctly answering 33 prompts while providing incorrect responses to 21 prompts, resulting in a hallucination percentage of 39%. Similarly, ChatGPT 4 demonstrated an overall success rate of approximately 72%, accurately answering 39 prompts while misguiding with 15 prompts, corresponding to a hallucination percentage of 28%.
Nonsensical Output
At times, LLMs can generate text that lacks logical coherence or meaningful content. This type of hallucination manifests as nonsensical output, where the generated text fails to convey any relevant or comprehensible information. Nonsensical hallucinations hinder the usability and reliability of LLMs when adopting the new technology in practical applications.
Irrelevant or Random LLM Hallucinations
LLMs may also generate hallucinations in the form of irrelevant or random information that holds no pertinence to either the input or the desired output. These hallucinations contribute to the generation of extraneous and potentially misleading content. Irrelevant or random hallucinations can confuse users and impair the usefulness of LLM-generated text.
By recognizing and understanding these types of hallucinations, we can better address the challenges and limitations of LLMs. Effective mitigation strategies can help improve the accuracy, coherence, and trustworthiness of LLM-generated responses across various domains.
Consequences of LLM Hallucination: Addressing Ethical Concerns
The phenomenon of hallucination in LLMs can have significant consequences, giving rise to several ethical concerns. By understanding these consequences, we can recognize the potential negative impacts on users and society at large. Let’s explore some of the most important ethical concerns stemming from LLM hallucination.
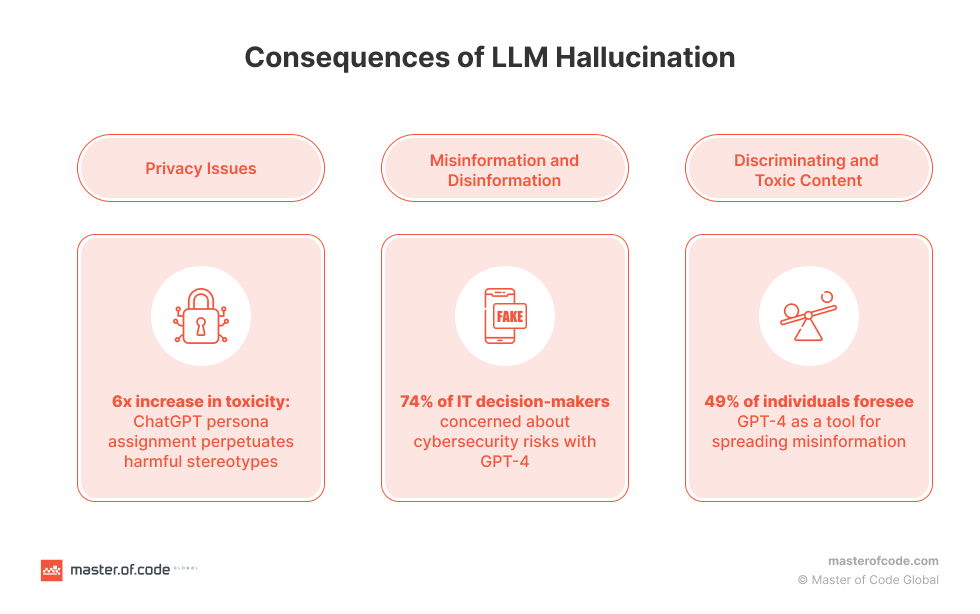
Discriminating and Toxic Content
LLMs have the potential to perpetuate and amplify harmful biases through hallucinations, giving rise to the production of discriminatory and toxic content. Recent research highlights the impact of persona assignment on ChatGPT, indicating that its toxicity can increase up to 6 times, resulting in outputs that endorse incorrect stereotypes, harmful dialogue, and hurtful opinions. The training data utilized for LLMs often contains stereotypes, which can be reinforced by the models themselves.
For instance, studies have revealed significant over-representation of younger users, primarily from developed countries and English-speaking backgrounds, within LLMs. Consequently, LLMs may generate discriminatory content targeting disadvantaged groups based on factors such as race, gender, religion, ethnicity, and more. For example, certain entities, such as specific races, are 3 times more targeted regardless of the assigned persona, indicating the presence of inherent discriminatory biases within the model. These hallucinated outputs have the potential to perpetuate harmful ideas, contributing to the marginalization and discrimination of vulnerable communities.
Privacy Issues
LLMs, with their training on extensive datasets, may raise concerns regarding privacy violations as they may contain personal information about individuals. Research findings from Blackberry indicate that an overwhelming 74% of IT decision-makers not only recognize the potential cybersecurity threat posed by GPT-4 but also express significant concern about its implications. Furthermore,according to recent ChatGPT and Generative AI statistics, approximately 11% of the information shared with ChatGPT by employees consists of sensitive data, including client information. Instances have occurred where LLMs inadvertently leaked specific personal details, such as social security numbers, home addresses, cell phone numbers, and medical information. These privacy breaches underscore the importance of implementing robust measures to safeguard personal data when deploying LLMs, considering the serious implications that can arise for individuals.
Misinformation and Disinformation
One of the critical consequences stemming from LLM hallucination is the generation of seemingly accurate yet false content lacking empirical evidence. Due to limited contextual understanding and the inability to fact-check, LLMs can inadvertently propagate misinformation, leading to the spread of inaccurate information. In a survey, approximately 30% of respondents expressed dissatisfaction with their GPT-4 experience, primarily due to incorrect answers or a lack of comprehension. Moreover, there is the potential for malicious intent, as individuals may intentionally exploit LLMs to disseminate disinformation, purposefully propagating false narratives. Notably, research conducted by Blackberry revealed that a significant 49% of individuals hold the belief that GPT-4 will be utilized as a means to proliferate misinformation and disinformation. The unchecked proliferation of misinformation and disinformation through LLM-generated content can have far-reaching adverse consequences across social, cultural, economic, and political domains.
Addressing these ethical concerns surrounding LLM hallucination is essential to ensure responsible and ethical use of these powerful language models. Mitigating biases, enhancing privacy protection, and promoting accurate and reliable information are crucial steps towards building trustworthy and beneficial LLM applications and AI chatbots.
Mitigating Hallucination in LLMs: Strategies for Organizations
To address the challenges posed by hallucination in LLMs, organizations integrating Generative AI, such as ChatGPT, can employ various strategies to minimize the occurrence of inaccurate or misleading responses.
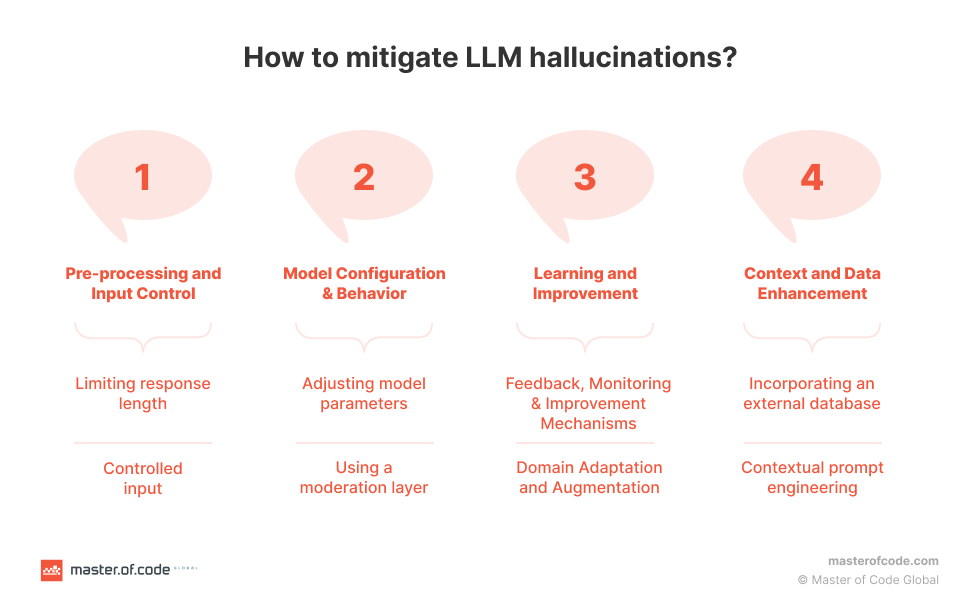
By implementing the following approaches, organizations can enhance the reliability, accuracy, and trustworthiness of LLM outputs:
Pre-processing and Input Control:
- Limiting response length: By setting a maximum length for generated responses, the chance of irrelevant or unrelated content is minimized. This helps ensure that the generated text stays coherent and facilitate personalized and engaging experiences for users.
- Controlled input: Instead of offering a free-form text box, providing users with specific style options or structured prompts guides the model’s generation process. This helps to narrow down the range of possible outputs and reduces the likelihood of hallucinations by providing clearer instructions.
Model Configuration and Behavior:
- Adjusting model parameters: The generated output is greatly influenced by various model parameters, including temperature, frequency penalty, presence penalty, and top-p. Higher temperature values promote randomness and creativity, while lower values make the output more deterministic. Increasing the frequency penalty value encourages the model to use repeated tokens more conservatively. Similarly, a higher presence penalty value increases the likelihood of generating tokens not yet included in the generated text. The top-p parameter plays a role in controlling response diversity by setting a cumulative probability threshold for word selection. Altogether, these parameters offer flexibility in fine-tuning and enable a balance between generating diverse responses and ensuring accuracy.
- Using a moderation layer: Implementing a moderation system helps filter out inappropriate, unsafe, or irrelevant content generated by the model. This adds an additional layer of control to ensure that the generated responses meet predefined standards and guidelines.
Learning and Improvement:
- Establish Feedback, Monitoring, and Improvement Mechanisms: To ensure the reliability of LLM outputs, organizations should implement robust evaluation, feedback, and improvement mechanisms. This includes engaging in an active learning process where prompt refinement and dataset tuning occur based on user feedback and interactions. Rigorous adversarial testing can also be conducted to identify vulnerabilities and potential sources of hallucination. Incorporating human validation and review processes, as well as continuously monitoring the model’s performance and staying updated with the latest developments, are crucial steps in mitigating hallucination and improving customer experiences.
- Facilitate Domain Adaptation and Augmentation: To enhance response accuracy and reduce hallucination, organizations can provide domain-specific knowledge to the LLM. Augmenting the knowledge base with domain-specific information allows the model to answer queries and generate relevant responses. Additionally, fine-tuning the pre-trained model using domain-specific data aligns it with the target domain, reducing hallucination by exposing the model to domain-specific patterns and examples.
Context and Data Enhancement:
- Incorporating an external database: Providing access to relevant external data sources during the prediction process enriches the model’s knowledge and grounding. By leveraging external knowledge, the model can generate responses that are more accurate, contextually appropriate, and less prone to hallucination.
- Employing contextual prompt engineering: Designing prompts that include explicit instructions, contextual cues, or specific framing techniques helps guide the LLM generation process. By providing clear guidance and context, GPT prompts engineering reduces ambiguity and helps the model generate more reliable and coherent responses.
These strategies collectively aim to reduce hallucination in LLMs by controlling input, fine-tuning the model, incorporating user feedback, and enhancing the model’s context and knowledge. By implementing these approaches, organizations can improve the reliability, accuracy, and trustworthiness of the generated responses.
Final Thoughts
LLM hallucination poses significant challenges in generating accurate and reliable responses, stemming from factors such as source-reference divergence, biased training data, and privacy concerns, leading to potential spread of misinformation, discriminatory content, and privacy violations. To mitigate potential risks, organizations can implement strategies such as pre-processing and input control, model configuration and behavior adjustments, learning and improvement mechanisms, and context and knowledge enhancement. These approaches help minimize hallucination and improve the quality and trustworthiness of LLM outputs.
In addition to these strategies, organizations can leverage innovative solutions like Master of Code’s LOFT (LLM Orchestration Framework Toolkit). LOFT offers a resource-saving approach that enables the integration of Generative AI capabilities into existing chatbot projects without extensive modifications. By seamlessly embedding LOFT into the NLU provider and the model, organizations can have greater control over the flow and answers generated by the LLM. LOFT provides users with the necessary data through external APIs and allows the addition of rich and structured content alongside the conversational text specific to the channel. By utilizing LOFT, organizations can effectively address concerns related to hallucination while maximizing the benefits of Generative AI technology.
By adopting these mitigation strategies and exploring tools like LOFT, organizations can navigate the challenges of LLM hallucination, ensuring the delivery of reliable and contextually appropriate responses. However, it is crucial to maintain an ongoing evaluation process and remain vigilant to address this complex challenge effectively and promote responsible AI practices.
Don’t miss out on the opportunity to see how Generative AI chatbots can revolutionize your customer support and boost your company’s efficiency.