Keeping machines running isn’t the challenge—it’s knowing exactly when and why to intervene before something breaks. For manufacturers under pressure to reduce costs, minimize downtime, and make smarter use of aging assets, the stakes are no longer just operational—they’re strategic. Yet most articles on AI-led maintenance barely move past surface-level theory or vendor hype. Odds are, you’ve rolled your eyes at it too: corporate speak that sounds big but says nothing.
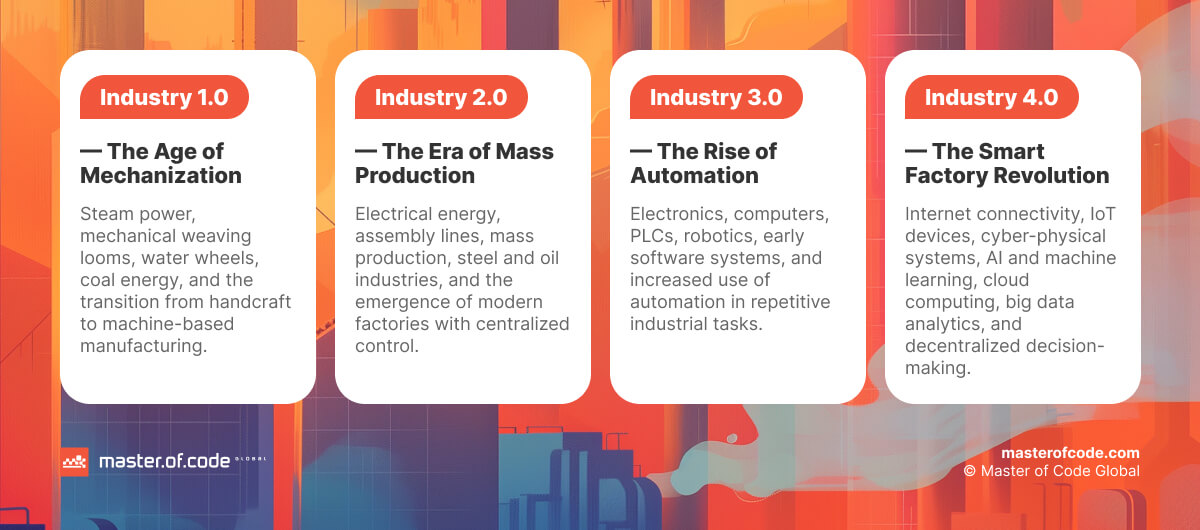
That’s exactly why we built this guide differently. Drawing from our hands-on experience designing and delivering fully custom intelligent solutions, we break down the mechanics of AI for predictive maintenance in manufacturing, show how it works inside real production environments, and map out the most impactful tactics in a structured way. You’ll also find real company examples—with results, not promises. Whether you’re looking to justify investment, improve planning, or scale smarter upkeep across sites, the answers start here.
Table of Contents
Predictive Maintenance as a Pillar of Industry 4.0
Proactive equipment upkeep isn’t just a technical upgrade—it’s a departure from the old logic of industrial operations. Traditionally, manufacturers relied on fixed maintenance schedules, visual inspections, or worst-case-scenario planning. The outcome? Some machines received unnecessary servicing, while others were left vulnerable—leading to unexpected breakdowns, bloated budgets, and production delays that ripple across the supply chain.
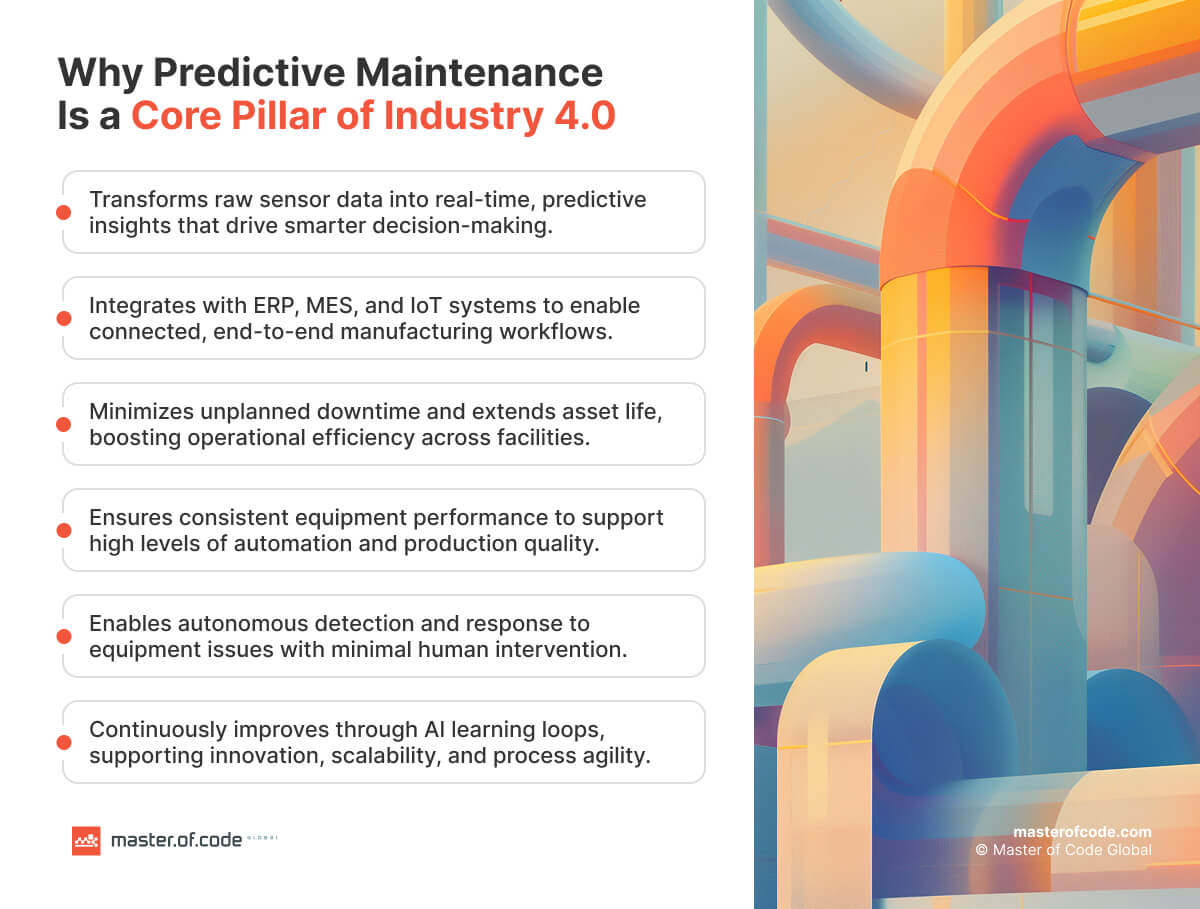
In contrast, AI-powered predictive models are flipping that approach entirely. Instead of reacting to failure or checking devices “just in case,” factories now receive accurate predictions derived from real-time data, historical patterns, and asset-specific conditions. According to the recent survey, 60% of manufacturers are actively moving away from reactive or time-based strategies. And among those that have adopted such systems, 88% report fewer breakdowns and improved asset visibility.
This metamorphosis isn’t about caring more—it’s about thinking differently. AI for predictive maintenance in manufacturing is repositioning infrastructure servicing from a reactive support task to an evidence-driven command center that protects uptime, maximizes asset output, and helps orchestrate production agility. By integrating intelligent monitoring across robotics, ERP, and connected machines, businesses are redesigning processes around foresight, not just response. This modification not only enhances equipment-level decisions but also contributes to broader outcomes like AI supply chain traceability, where machinery health data feeds into end-to-end visibility.
Let’s unpack what powers this shift—and how it works in action.
What Is AI-Driven Predictive Maintenance in Manufacturing?
Think of it as giving your machines a sixth sense. Instead of waiting for signs of wear or relying on fixed service intervals, manufacturers can now anticipate risk long before it derails operations. This approach employs advanced analytics to move upkeep from reactive firefighting to foresight-driven strategy.
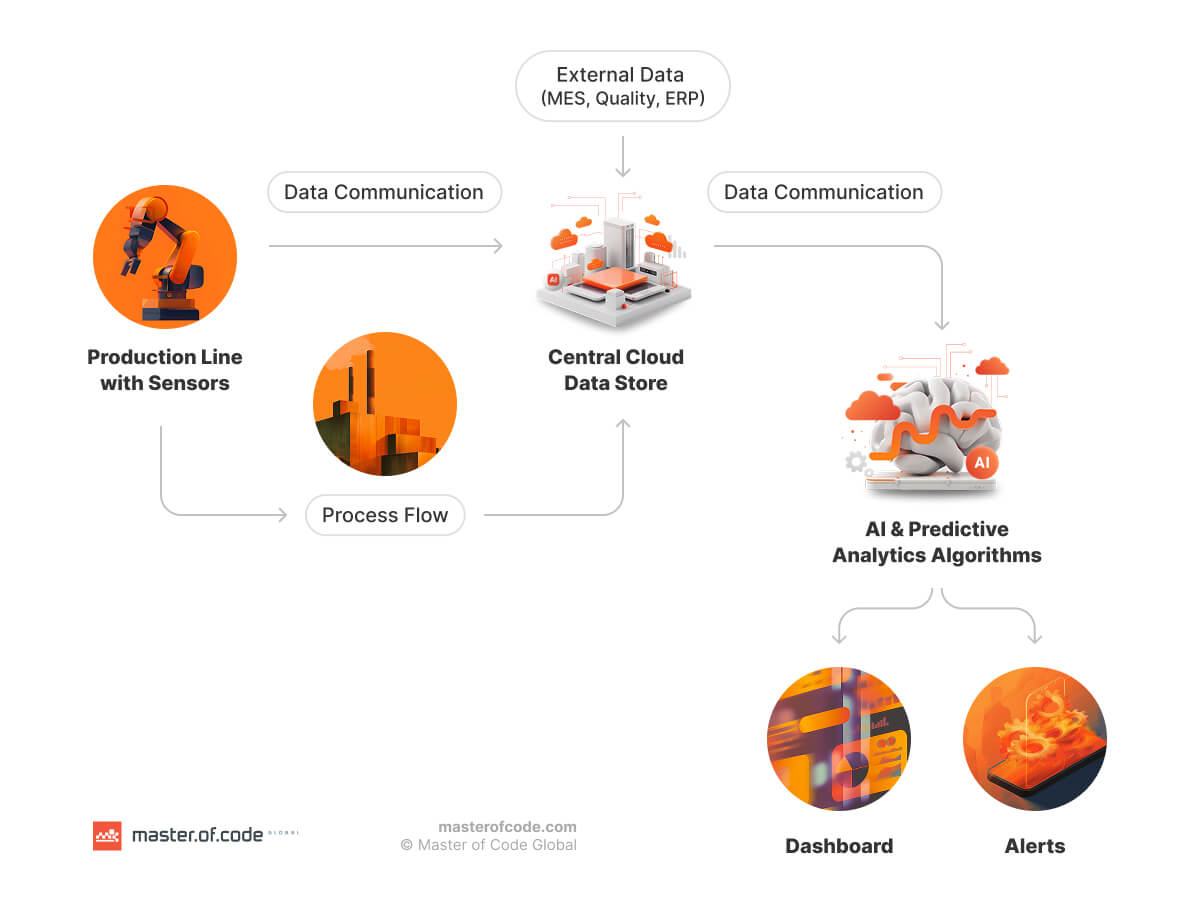
Unlike reactive maintenance, which waits for things to break, or preventative maintenance, which risks over-servicing, this model uses patterns in sensor readings, performance metrics, and historical data to determine when intervention is truly needed. The result? Fewer unplanned shutdowns, lower risk of machine failure, and smarter use of resources.
At its core, predictive maintenance in manufacturing using AI empowers teams to take action not because the clock says so—but because the data does. But how exactly does AI make that call? Let’s look at the mechanisms that drive this decision-making process.
Inside the Engine: How Intelligent Predictive Maintenance Works
At the core of this approach is a highly coordinated system of information gathering, pattern recognition, and decision automation. Each element plays a distinct role in shifting upkeep from assumption-based to intelligence-led.
- Sensor data analysis. Modern manufacturing equipment is embedded with IoT sensors that continuously track and decipher metrics like temperature, vibration, pressure, and acoustic signals. These inputs serve as indicators of asset health, capturing subtle shifts that would otherwise go unnoticed.
- Data aggregation. Sensor readings are funneled into centralized platforms—often powered by cloud or edge computing—where they’re synchronized and stored. The unified dataset facilitates end-to-end visibility and paves the way for cross-machine comparisons.
- Historical data & baselining. To detect anomalies, AI applications first need to understand what “normal” looks like. By analyzing the legacy records, models learn baseline operating conditions unique to each asset, accounting for variables like workload, age, and usage patterns.
- Deviation identification. Employing AI anomaly detection, the system reports deviations from established baselines, such as increased vibration or abnormal heat signatures. Such early signs can be a clear warning that a machine failure is imminent.
- Predictive modeling. Once malfunctions are identified, advanced algorithms estimate the likelihood and timing of component failure. This enables proactive interventions that are both timely and cost-effective, reducing risk without unnecessary downtime.
- Automated maintenance schedules. Based on risk assessments, AI systems can initiate work orders or adjust plans dynamically. This ensures service happens at the most optimal window, avoiding disruption while extending asset lifespan.
- Tools and tech. Key technologies include ML-driven platforms, digital twins for real-time simulation, cloud analytics for consolidated insights, edge devices for local processing, and diagnostic dashboards that visualize condition at a glance.
Thus, AI-powered predictive maintenance in manufacturing follows a logical chain: data is collected, analyzed for patterns and anomalies, used to predict upcoming failures, and then translated into optimized maintenance schedules. The real impact lies not just in early warnings, but in aligning those insights with actions that minimize downtime and allocate resources more strategically.
Now that we’ve broken down the technical foundation, let’s explore the various strategies manufacturers can adopt—and where technology brings the most value.
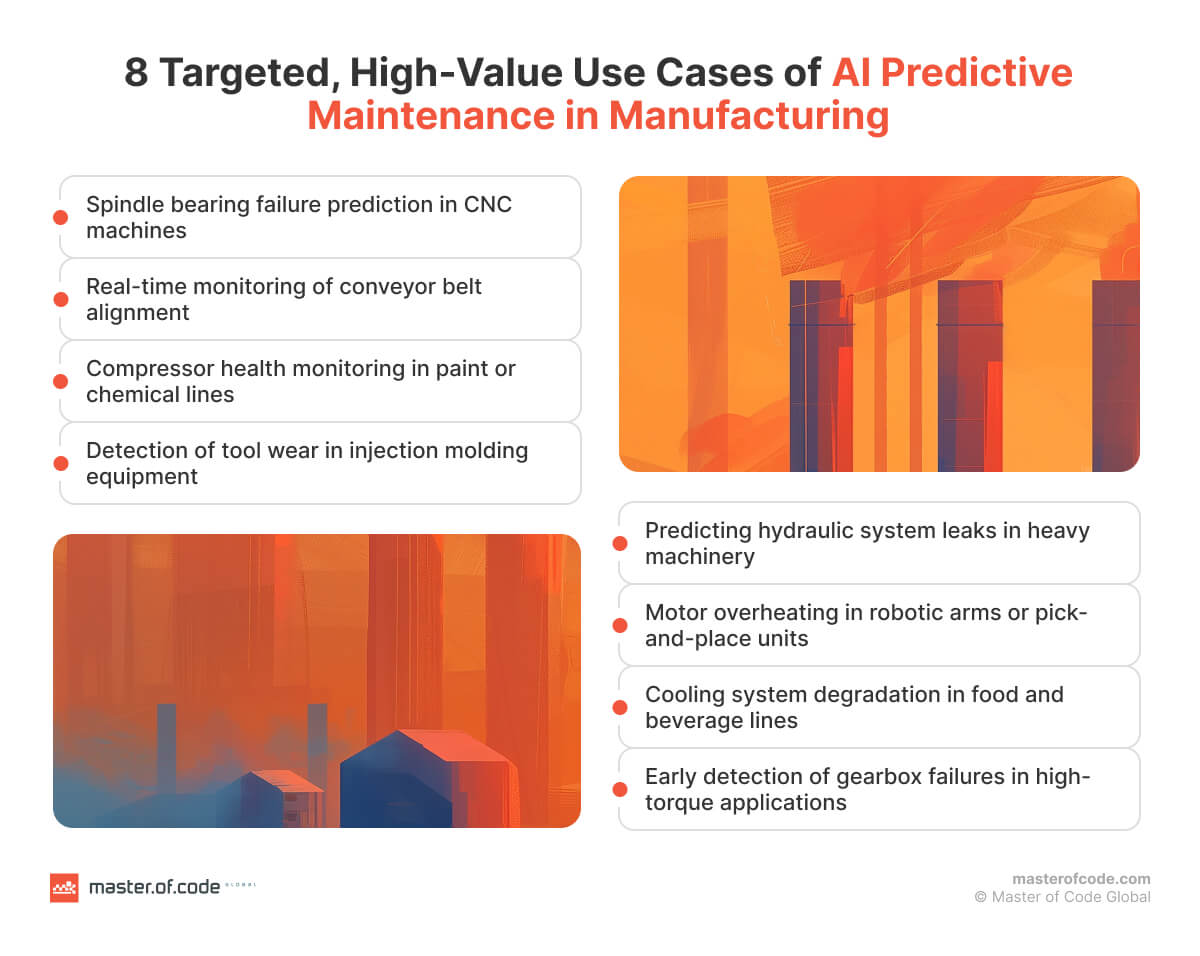
AI Predictive Maintenance Tactics Tailored to Different Equipment Classes
Not all machines require the same approach. The type of asset, its function, and operational risk all influence how AI-driven predictive maintenance in manufacturing is applied across the factory floor. Below are the most common and impactful categories.
1. Predictive Maintenance for Industrial Equipment
Tracks large-scale machinery like motors, turbines, and compressors using real-time sensor data. Machine learning detects anomalies and predicts failures before they disrupt production.
Why it’s crucial:
- Lowers emergency repair costs and spare part usage;
- Helps balance energy consumption during heavy-duty cycles;
- Enables better long-term capital planning for asset replacement.
2. Predictive Maintenance for Rotating Machinery
Covers pumps, gearboxes, and fans. AI analyzes vibration patterns, speed shifts, and acoustic emissions to detect early signs of wear or misalignment.
Why it’s crucial:
- Increases lubrication efficiency and part longevity;
- Supports quieter, safer machine operation;
- Reduces downstream strain on connected systems.
3. Predictive Maintenance for CNC and Precision Tools
AI defect detection monitors equipment like CNC mills, lathes, and 3D printers. Algorithms then evaluate tool wear, cutting force, and thermal behavior to prevent tolerance drift or item imperfections.
Why it’s crucial:
- Makes sure tool life is fully utilized without risking part accuracy;
- Allows consistent product quality across long manufacturing cycles;
- Improves scheduling by minimizing unexpected changes.
4. Predictive Maintenance for Conveyor and Material Handling Systems
Gathers load, speed, and tension data from conveyors, lifts, and AGVs. AI flags issues before they cause flow interruptions or mechanical stoppages.
Why it’s crucial:
- Strengthens synchronization between automated handling units;
- Decreases wear on belts and motors under variable loads;
- Boosts energy efficiency in high-throughput operations.
5. Predictive Maintenance for Robotic Systems
Tracks torque, motor health, and alignment in robotic arms and cobots. AI identifies degradation before it affects motion accuracy or safety.
Why it’s crucial:
- Minimizes idle period caused by manual diagnostics;
- Upholds compliance in safety-critical or regulated environments;
- Empowers systems to calibrate in advance for consistent operations.
Want to go deeper? In the next section, we’ll explore the enterprise-level advantages that make these AI strategies not just smart—but profitable.
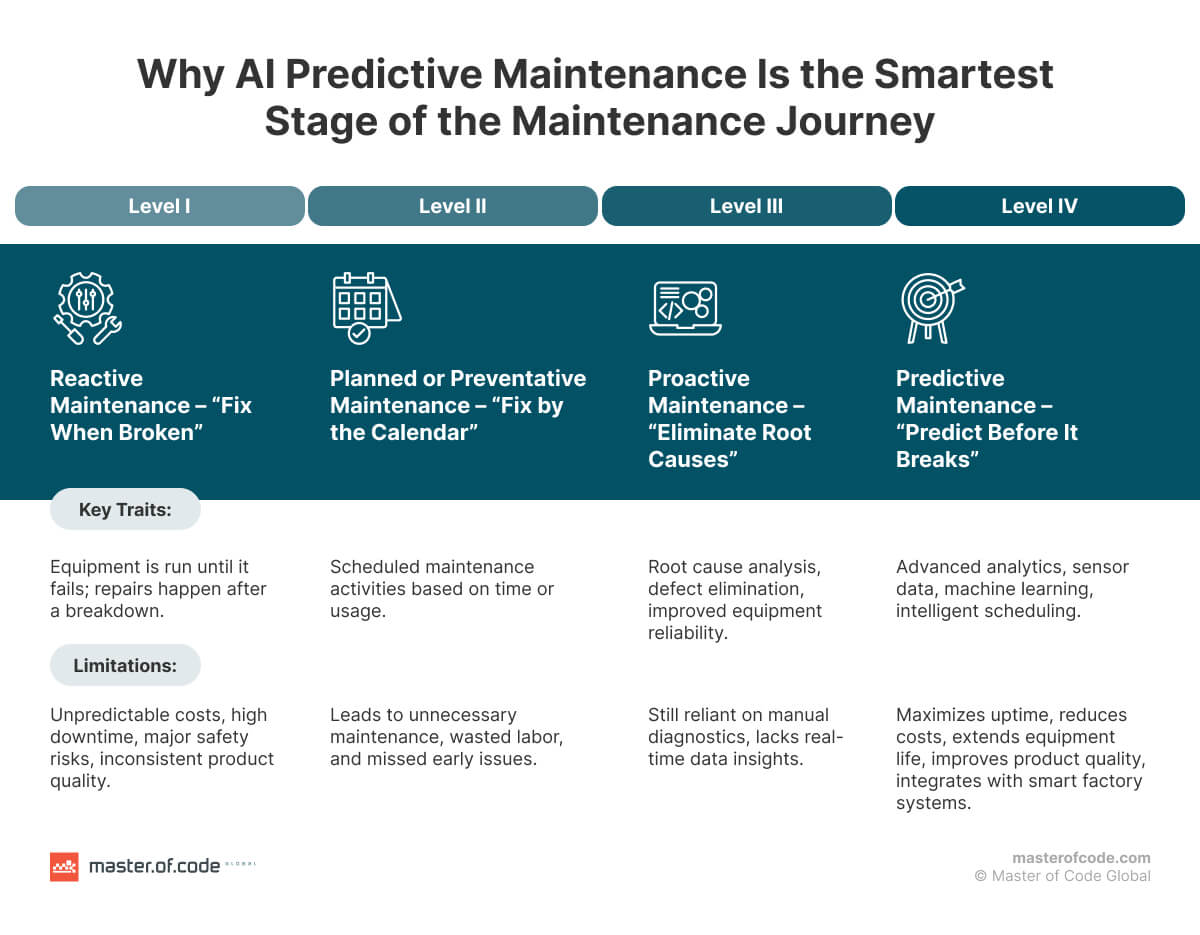
From Cost Drain to Cost Saver: The Business Benefits of Intelligent Predictive Maintenance
The impact of AI in predictive maintenance manufacturing is already visible on factory floors. Manufacturers report gains in planning accuracy, cost control, and operational agility—well beyond failure prevention. And with 43% identifying production operations as the main area to benefit, the technology is quickly becoming essential to how modern plants run, scale, and compete.
Strategic Advantage | Quantified Impact |
---|---|
Lower operational and servicing expenses | 30% avg. reduction in maintenance costs and 10% in spare parts costs |
Cutbacks in processes and resources | 5–10% cost savings in operations and MRO material spend |
Increased asset uptime and production continuity | 10–20% boost in machine uptime and availability |
Streamlined long-term servicing approach and resource usage | 20–50% reduction in maintenance planning efforts |
Improved reliability and fewer malfunctions | 70% reduction in equipment breakdowns on average |
Higher workforce efficiency | 5–20% increase in labor productivity |
Greater facility throughput and capacity utilization | 5–15% reduction in downtime, freeing up capacity |
Better inventory turnover with lower storage burden | 10–30% drop in inventory levels and 5–20% in carrying costs |
Delayed capital expenditure on new equipment | 3–5% lower spending on new tools |
These numbers don’t just highlight efficiency gains—they reveal how machine learning is reshaping the value manufacturers extract from their assets. Let’s now take a closer look at how these capabilities play out in more advanced, real-world scenarios.
Advanced Applications of AI for Predictive Maintenance
Artificial intelligence isn’t just changing how servicing is done—it’s altering where, when, and why it’s done. Below are AI predictive maintenance manufacturing examples that showcase how different companies apply smart upkeep strategies across operational environments.
1. Dynamic Workflow Automation
AI-driven predictive systems can autonomously adjust maintenance schedules and reroute workloads based on real-time insights. This dynamic approach guarantees optimal resource utilization and minimizes downtime.
Real-world example: Nestlé has embedded predictive technology into its factory automation strategy to dynamically modify operations and simplify workflows. The results are reduced interruptions, increased agility, and flexible production at scale.
2. Real-Time Alerts and Escalation
Systems powered by machine learning can trigger immediate alerts or initiate maintenance protocols autonomously upon detecting critical equipment issues. Consequently, rapid response capability minimizes the risk of breakdowns and delays. This is exactly the kind of outcome that validates why AI predictive maintenance prevents unplanned downtime in the first place.
Real-world example: BlueScope Steel integrated a GenAI-fueled upkeep solution to streamline tools monitoring. With real-time warnings and recommendations, it boosts reaction speed and supports less-experienced teams on the shop floor.
3. Smart Part Ordering
AI forecasts maintenance needs and aligns parts inventory or supplier timelines accordingly, making sure necessary components are available when needed. This proactive approach reduces expenses and prevents delays. In sectors that rely on AI in fleet management, it helps synchronize part availability with predictive intervals across moving assets.
Real-world example: Though not exactly manufacturing, GE Aerospace uses AI to monitor 44,000 in-service engines and detect issues before failure occurs. Real-time alerts help maintenance teams act early and cut lifecycle costs. The same principle can apply to manufacturers looking to tie equipment data to part ordering for smarter inventory planning.
4. Cross-Factory Optimization
Maintenance systems applied across multiple sites learn from patterns to optimize performance globally. This holistic approach advances operational efficiency and standardizes best practices—especially when deployed alongside AI supply chain predictive maintenance, where asset insights extend across plants, warehouses, and supplier networks.
Real-world example: Makino Asia connects its machining and assembly plants through IIoT-based predictive maintenance. By monitoring machine health across sites in real time, it prevents breakdowns, optimizes servicing, and enables consistent output across its smart factory network.
5. Early Fault Detection
Maintenance systems continuously monitor equipment parameters to identify subtle anomalies that precede failures. By analyzing patterns in sensor data, these solutions can detect issues like minor vibrations or temperature fluctuations, enabling teams to address problems before they escalate, like machine failure.
Real-world example: BMW Group’s Regensburg plant implemented an AI-supported system to observe the conveyor during vehicle assembly. The software detects irregularities and automatically alerts technicians, helping avoid over 500 minutes of unplanned downtime annually.
FAQ: Quick Answers to Key Questions
What are the benefits of using AI for predictive maintenance in manufacturing?
Predictive maintenance helps reduce unplanned downtime, raise asset reliability, and extend equipment lifespan. It also enables more efficient use of resources by aligning upkeep efforts with real-time machine conditions.
How does AI-driven predictive maintenance work in manufacturing?
Employing machine learning, the system analyzes sensor data to identify patterns and detect anomalies. These insights help predict when equipment is likely to fail, allowing teams to plan servicing before issues escalate.
How does Generative AI improve predictive maintenance in manufacturing?
GenAI adds a new layer of decision support by transforming complex data into clear, contextual recommendations. It accelerates response times, streamlines troubleshooting, and sustains knowledge sharing across teams.
Final Thoughts: Predict Before You Repair
AI for predictive maintenance in manufacturing isn’t a future-state—it’s already reshaping how leading factories run smarter, leaner, and with fewer surprises. But reading about it and doing it are two very different things.
That’s where experience makes the difference. At Master of Code, we’ve spent over 20 years helping enterprises move from reactive operations to data-led decision-making. With over 1,000 projects delivered and applications deployed across industries, we’ve learned what works—and, more importantly, what doesn’t. Moreover, our partnerships with Salesforce, AWS, Google, and others let us build faster, smarter, and more scalable AI predictive analytics solutions 100% tailored to your environment.
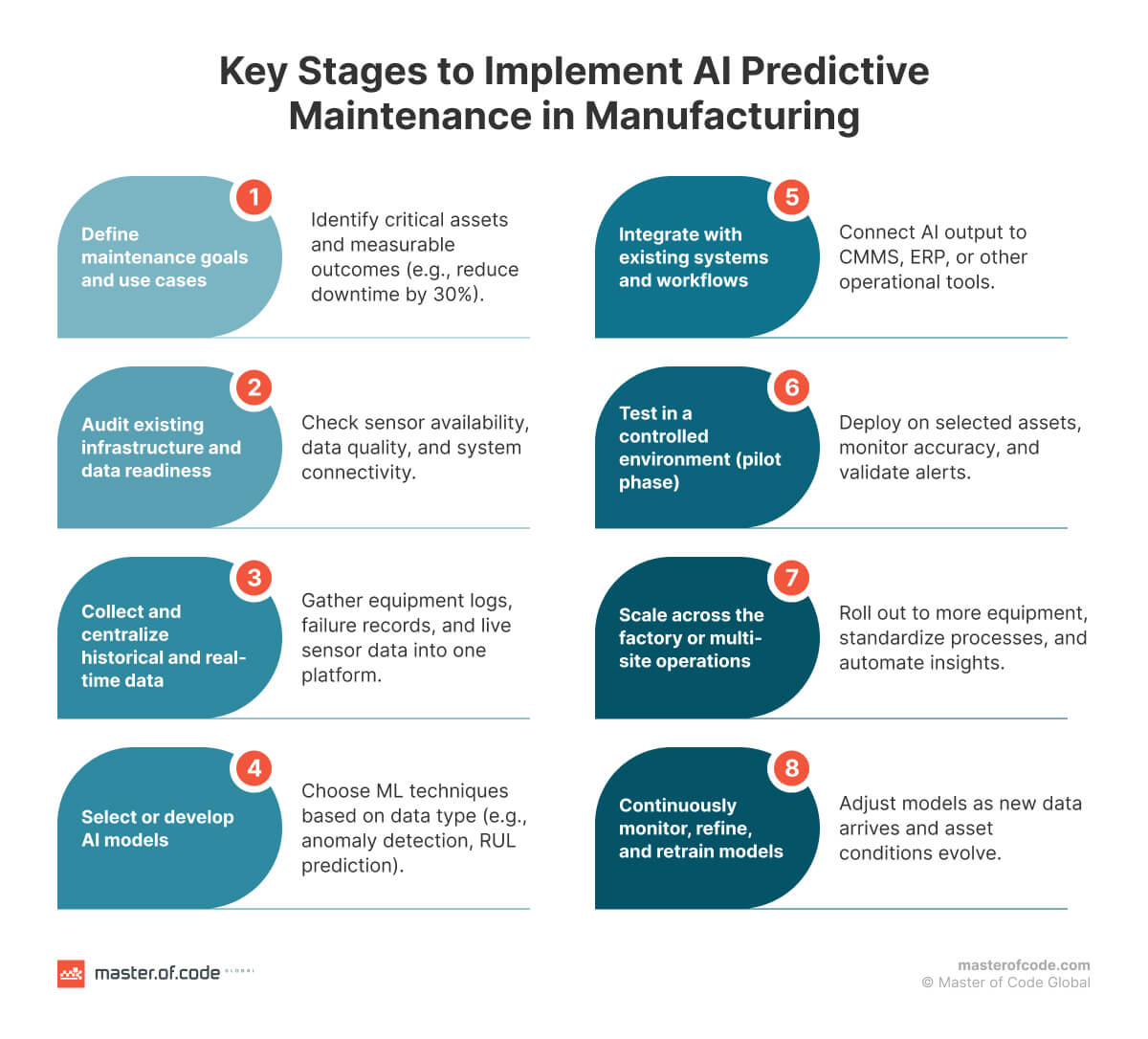
So, where should you begin? Based on our proven best practices, here’s a reliable starting roadmap:
- Assess asset readiness and data maturity. Define what can be monitored and where the gaps are.
- Prioritize use cases with the highest ROI. Focus on areas where downtime is most costly or visibility is lowest.
- Develop a pilot with tangible outcomes. Start small but ensure real-time data, prediction accuracy, and scheduling integration.
- Scale across assets and sites. Once the value is validated, standardize and expand with learnings from the pilot.
In the end, execution is what separates pilot projects from long-term impact. Choosing the right partner turns potential into performance. If you’re ready to explore how AI predictive maintenance can drive measurable results in your operations, let’s talk. We’ll show you exactly what’s possible—and what’s profitable—for your specific business. For us, personalization isn’t a tagline—it’s the foundation of every solution we deliver.
Ready to build your own Conversational AI solution? Let’s chat!