Once upon a time, there existed a medical organization that appeared magnificent from the outside. Its modern facilities gleamed, its reputation soared, and clients arrived daily seeking healing. Yet behind this impressive facade, the kingdom struggled with a hidden challenge: mountains of untapped data scattered across disconnected realms.
Clinical notes, billing details, and patient records accumulated in vast, isolated repositories. Physicians made decisions based on limited information, while administrators wrestled with resource allocation without seeing the complete picture. The kingdom’s greatest treasure—its data—remained largely unused, locked away in separate towers.
This story might sound like fantasy, but it reflects the reality of many institutions today. The exception? Forward-thinking organizations that stay informed through our blog, where we regularly explore existing challenges and their innovative solutions. These industry leaders have already discovered AI predictive analytics in healthcare as the answer to their data dilemmas. The results speak for themselves: disease risks identified earlier, patient outcomes predicted with remarkable accuracy, and resources allocated with unprecedented precision.
On top of that, by relying on our fully tailored predictive AI services, these visionary care providers gain additional competitive advantages. They’re not just forecasting admissions; they’re preventing them. They’re not merely personalizing treatment plans; they’re optimizing them. And they’re not simply reducing complications; they’re anticipating and eliminating them entirely. Their ecosystems have evolved beyond reactive decision-making to a state of continuous, data-driven optimization that competitors struggle to match.
Is your business drowning in data yet starving for insights too? Join the leaders who’ve solved this paradox. Continue reading to discover how predictive AI in healthcare empowers smarter, forward-looking strategies.
Table of Contents
The Six Realms of Healthcare Data: Foundations of Forecasting Technology
In every medical ecosystem, six distinct territories of information exist—each containing unique insights. When combined through AI-driven predictive analytics in healthcare, they bring meaningful knowledge to light that once seemed impossible to obtain.
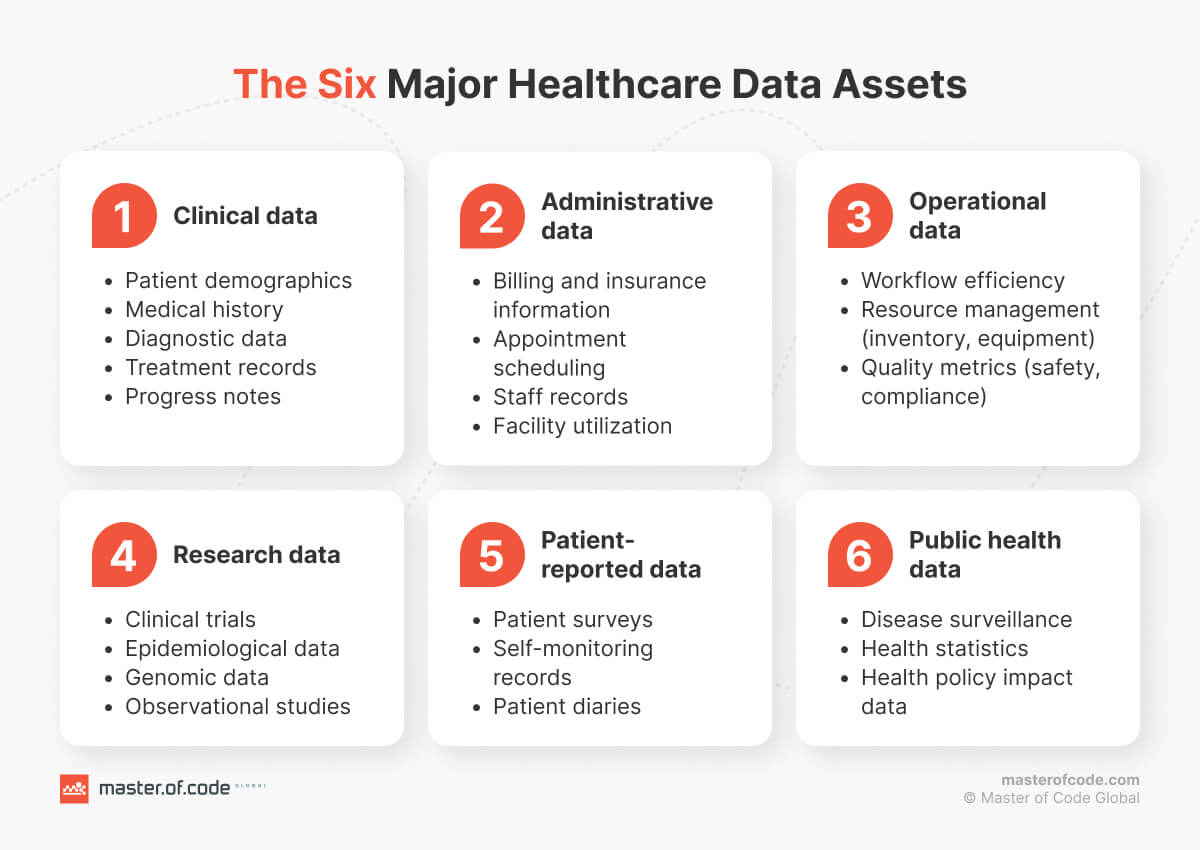
- The territory of Clinical Data houses the chronicles of individual journeys: client histories documenting past ailments, diagnostic results revealing hidden conditions, and therapy records capturing recovery paths. When properly utilized, these assets facilitate remarkable advancements in disease detection and the creation of effective treatment plans.
- Within Administrative Data lies the organization’s logistical wisdom: billing history, patient admission logs, and scheduling details. Such seemingly mundane documents contain valuable conclusions about operational efficiency and healthcare costs that transform chaotic processes into streamlined pathways.
- The Patient-Reported Data type contains first-hand accounts from those receiving service—their symptoms, experiences, and personal journals. To craft truly personalized treatment approaches addressing each person’s distinctive needs, healthcare providers rely on these authentic narratives for essential context.
- Research Data represents the collected expertise of practitioners—clinical trials, genomic discoveries, and epidemiological patterns. When this knowledge merges with AI-powered predictive analytics for healthcare, it accelerates scientific breakthroughs and enhances patient safety protocols.
- The Operational Data domain tracks the inner workings of facilities—workflow productivity, supply management, and quality metrics. Smarter resource allocation decisions—guided by such findings—enable organizations to optimize performance without compromising patient care.
- Finally, Public Health Data oversees population-wide patterns—tracking disease outbreaks, vaccination campaigns, and community wellness trends. This broader evidence helps protect entire communities from emerging threats while improving health outcomes.
When these six domains combine through advanced analytics, the landscape transforms from reactive to proactive, unlocking the true potential of modern medicine. But how exactly does this occur? Let’s examine the sophisticated tools that turn raw information into actionable foresight.
The Wizard’s Toolkit: How AI Predictive Analytics in Healthcare Works Its Magic
Beneath the impressive results of such insights lies a powerful toolbox with remarkable abilities to see the future through today’s medical lens. Traditional approaches functioned like statistical forecasting—relying on established patterns and visible indicators to make predictions. These conventional methods served the domain effectively for decades but struggled when confronted with the vast, intricate tapestry of modern patient data.
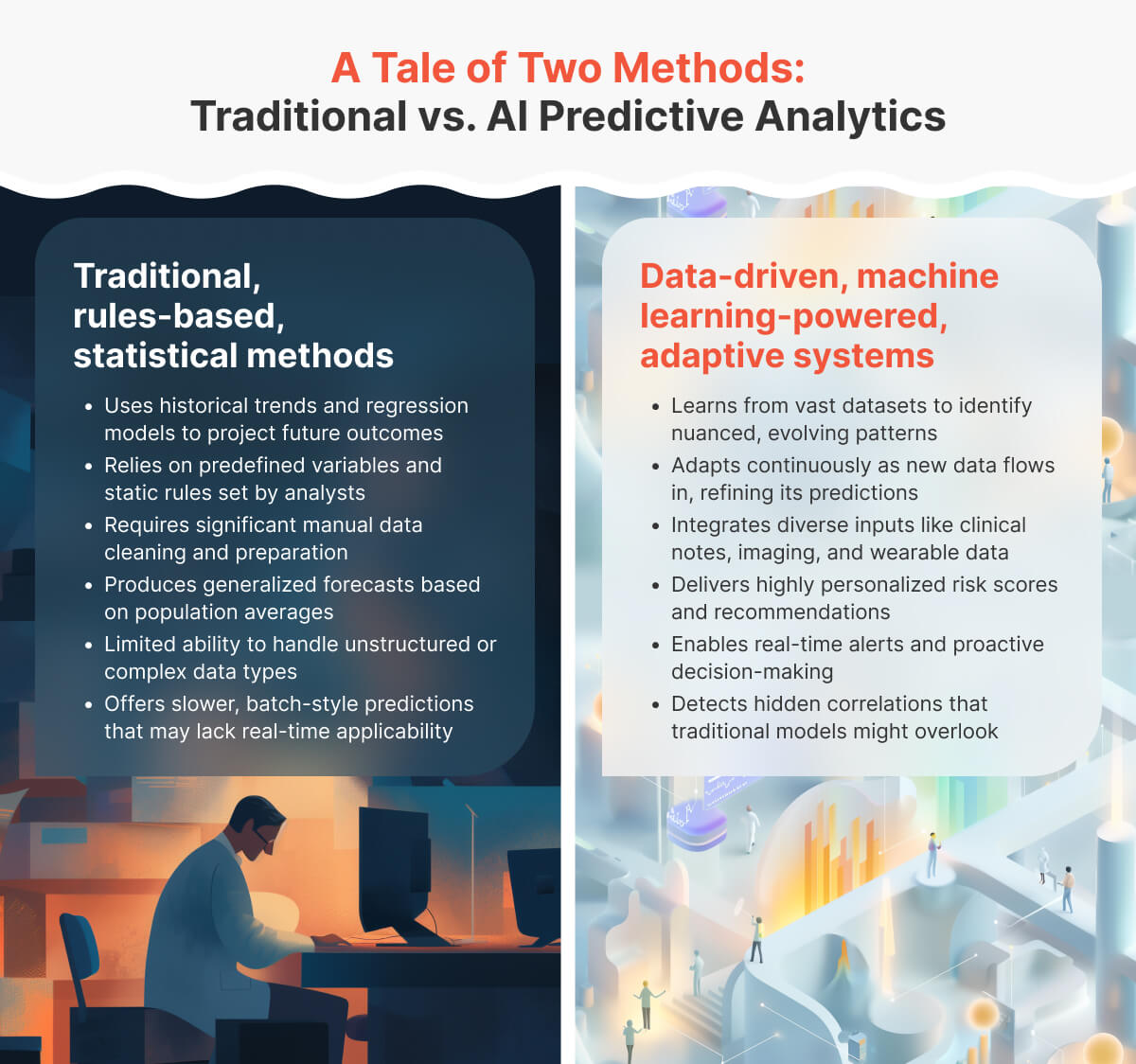
With the integration of AI predictive analytics for healthcare, everything changed. Intelligent algorithms pinpoint trends autonomously, becoming more effective through experience. Unlike their predecessors, this evolved technology can process thousands of variables simultaneously, discovering hidden connections human analysts might miss.
At the heart of this transformation are:
- Neural Networks, mirroring actual brain function, recognize nuanced links across heterogeneous sources. They facilitate precise forecasting from multidimensional data points.
- Machine Learning is self-improving algorithms. This means they can continuously refine their functionalities through exposure to new information.
- Natural Language Processing extracts meaningful insights from unstructured text in clinical notes, medical literature, and care documentation.
- Computer Vision offers advanced image analysis powers that detect minute abnormalities in imaging that human eyes easily overlook.
- Deep Learning analyzes extensive datasets to identify subtle indicators of future health events, particularly invaluable for complex treatment plans.
- Federated Learning allows models to learn across multiple systems while maintaining the privacy and security of sensitive details.
The real breakthrough happens when these systems bridge previously isolated repositories of knowledge. By connecting separate evidence types, AI in predictive healthcare analytics creates a comprehensive view of each individual’s health journey. The result is 100% personalized treatment guidance and optimized resource distribution.
Now, let’s explore four key application areas where this technology creates tangible value across the medical ecosystem in detail.
Quests and Conquests: AI Predictive Analytics in Healthcare Use Cases and Their Rewards
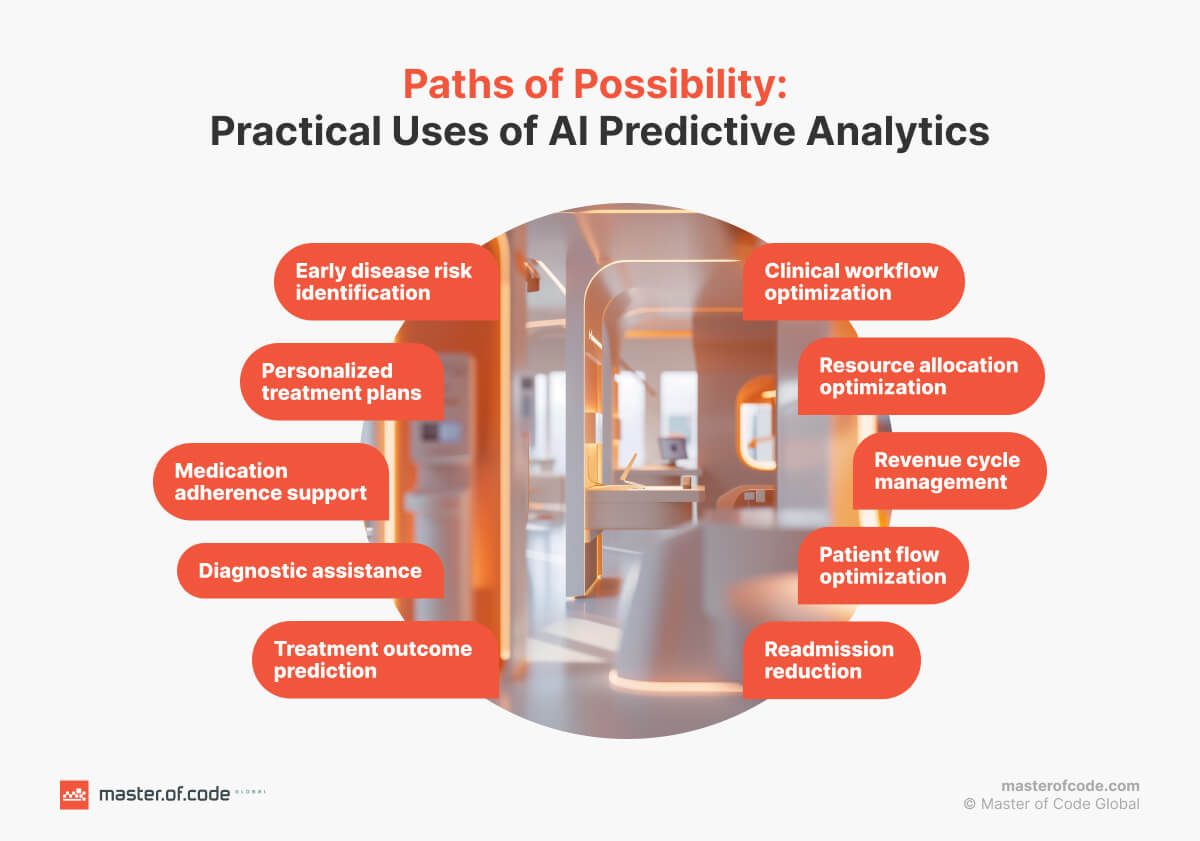
For the Citizens: Personalized Patient Care Through Prediction
#1: Early disease risk identification
Advanced intelligent models now analyze health data to identify illness susceptibility years before symptoms appear. In fact, studies showing 90%+ accuracy in forecasting conditions like cardiovascular disease:
- Predicting future health hazards by determining deviations and trends in an individual’s history.
- Combining different variables like genetics, lifestyle, and environmental factors to provide a detailed risk profile.
- Identifying subtle, pre-symptomatic indicators that may suggest the onset of a disease.
Benefits:
- Prevention rather than treatment.
- Lower out-of-pocket healthcare costs.
- Alleviated anxiety through proactive care.
- Better overall health outcomes.
#2: Personalized treatment plans
Algorithms powered by AI analyze each customer’s profiles to develop tailored therapeutic approaches. Clinical studies demonstrate these frameworks boost care outcomes by 30–35% compared to traditional methods.
- Informing decisions built on an individual’s unique biological and genetic characteristics.
- Forecasting how a person might respond to treatment changes and suggesting optimal adjustments.
- Predicting the likely course of a disease and potential outcomes for a specific client.
Benefits:
- Higher treatment effectiveness.
- Fewer adverse reactions.
- Shorter recovery times.
- Treatment matched to individual needs.
#3: Medication adherence support
How can we ensure patients consistently take their prescribed medications? Research shows AI-driven forecasting approaches can increase adherence rates by up to 30% in some cases by:
- Pinpointing individual habits that make it difficult to adhere to the prescription.
- Adjusting reminders as per the demonstrated obedience patterns.
- Connecting customers with relevant support networks based on their predicted adherence problems.
Benefits:
- Fewer complications from missed medications.
- Hospital readmissions prevention.
- Better chronic disease management.
- Higher quality of life.
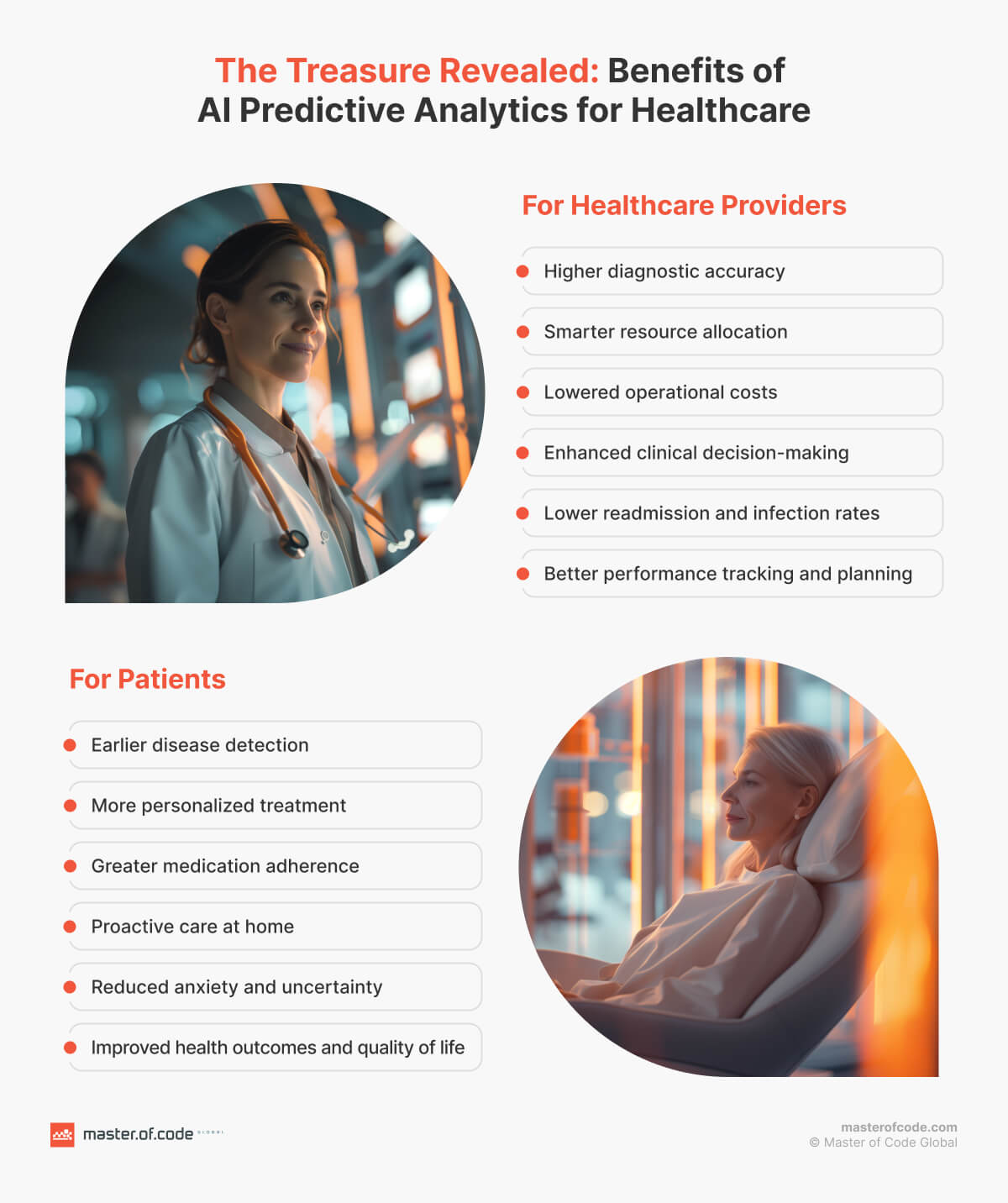
For the Healers: Enhanced Clinical Decision Support
#1: Diagnostic assistance
AI predictive analytics in healthcare have demonstrated diagnostic precision rates exceeding 90% across multiple specialties, helping physicians in several ways:
- Analyzing medical images (X-rays, MRIs) to detect subtle anomalies that might escape the human eye.
- Processing symptoms and history to suggest likely diagnoses and differential options.
- Highlighting relevant information from medical literature to support clinical reasoning.
Benefits:
- Higher diagnostic accuracy.
- Cut down on time to diagnosis.
- Identification of rare conditions.
- Decreased diagnostic testing costs.
#2: Treatment outcome prediction
Want to forecast reactions before selecting treatments? You can upgrade predictive analytics in healthcare using AI and limit uncertainty in decisions. For example, AI models can predict responses to immunotherapy with accuracy rates of 70–90%, depending on the specific approach.
- Forecasting potential response to various therapeutic options based on their individual profile.
- Anticipating the chances of complications or adverse events associated with different interventions.
- Estimating the likelihood of achieving specific health goals (e.g., remission, functional modification).
Benefits:
- Better informed treatment selection.
- Reduced trial-and-error approaches.
- More confident clinical decision-making.
#3: Workflow optimization and proactive maintenance
Artificial Intelligence can assist in addressing staffing challenges by performing up to 30% of administrative tasks that typically fall to healthcare providers, freeing them for direct care. Additionally, AI for predictive maintenance of medical devices can predict equipment malfunctions before they occur, preventing disruptions during critical procedures.
- Predicting patient flow and potential bottlenecks to optimize scheduling and resource allocation.
- Identifying customers prone to deterioration to prioritize timely interventions.
- Automating organizational tasks based on predicted requirements (e.g., pre-authorizations, follow-ups).
- Forecasting staffing needs derived from anticipated customer volume and acuity.
Benefits:
- Diminished administrative burden.
- More time for patient care.
- Prioritization of high-risk clients.
- Decreased provider burnout.
For the Grand Fortress: Hospital-Wide Transformation Through Prediction
#1: Patient flow optimization
Hospital AI predictive analytics, like skilled traffic controllers, analyze arrival patterns and resource availability to ensure a smoother journey for every customer.
- Analyzing historical and real-time patient data on arrivals and departures.
- Modeling people’s movement through different hospital departments.
- Predicting bed availability based on patient admissions, discharges, and acuity.
- Identifying bottlenecks in key transition points like the ER and operating rooms.
Benefits:
- Prevention of emergency department crowding.
- More streamlined admission and discharge processes.
- Better coordination between units.
- Enriched customer experience.
#2: Readmission reduction
Unplanned hospital readmissions cost the US healthcare system $17–$26 billion annually. By adopting AI in electronic health records and ML predictive models, organizations can minimize rehospitalization rates by 15–20%.
- Predicting individuals likely to be readmitted as per their medical history, release conditions, etc.
- Triggering proactive interventions and support for vulnerable individuals post-discharge.
- Pinpointing key factors contributing to readmissions to inform quality improvement initiatives.
Benefits:
- Lower readmission penalties.
- Better post-discharge coordination.
- Boosted quality metrics.
- Enhanced reputation.
#3: Hospital-acquired infection (HAI) prevention
HAIs affect approximately 1 in 31 hospitalized people. AI in health data analysis has demonstrated significant potential, with one ML model achieving 72% accuracy in forecasting urinary tract infections before symptoms appear.
- Identifying environmental or procedural factors that may contribute to infection spread.
- Monitoring patient data for early signs of illness to enable swift intervention.
- Predicting high-risk units or populations for specific types of infections.
- Optimizing hygiene protocols and resource allocation based on predicted disease susceptibility.
Benefits:
- Decreased infection rates.
- Lower treatment costs.
- Shorter stays.
- Improved patient outcomes and safety metrics.
Chronicles of Success: 6 Proven AI Predictive Analytics in Healthcare Examples
The theoretical promise of artificial intelligence has evolved into a practical reality across forward-thinking medical centers. Below, we examine how specific healthcare facilities have deployed AI in hospital management. They solve persistent problems—not just incrementally improving metrics, but fundamentally reimagining how decisions are made, resources are allocated, and care is delivered.
Master of Code Global’s AI Pharmacy Solution
Our team developed a sophisticated medication management solution for a major Canadian insurer. The platform employs advanced AI algorithms to provide drug interaction analysis by considering a user’s medical history and real-time research. It also offers proactive refill control by analyzing consumption patterns to ensure users never run out of essential medications.
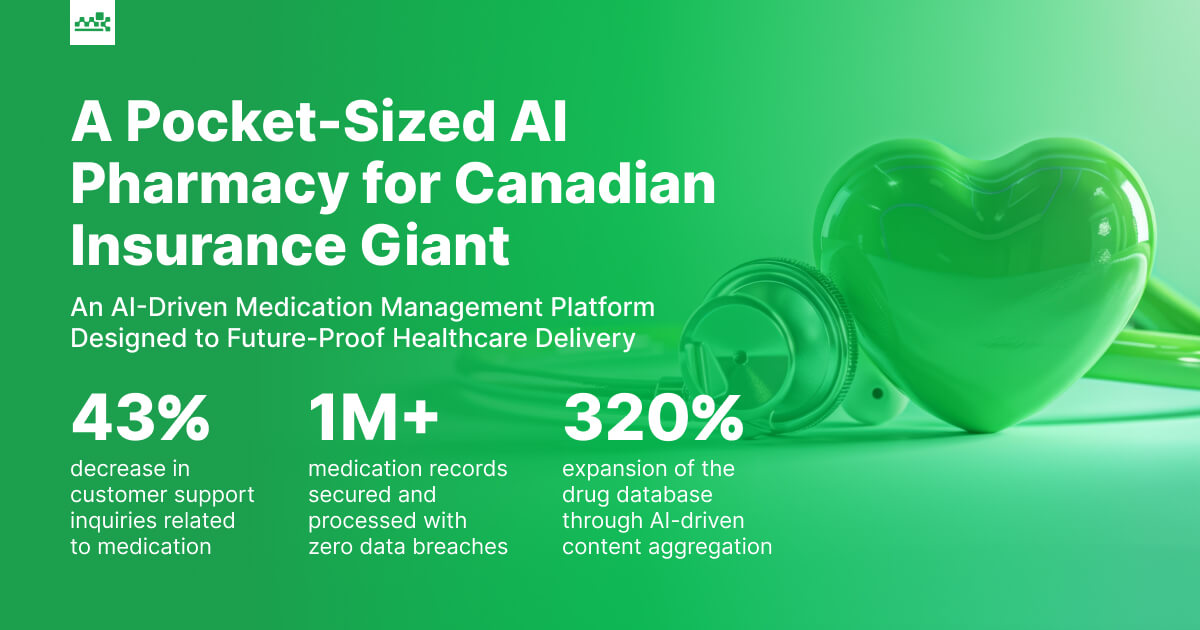
NYU Langone Health’s Readmission Oracle
The organization designed NYUTron, an AI software that examines physicians’ notes using LLM to predict patient outcomes. This “AI doctor” can accurately estimate the chances of rehospitalization within a month, achieving an 80% prediction rate. By learning directly from unstructured text in electronic records, NYUTron offers a significant improvement over traditional models.
Cleveland Clinic’s Risk Calculator
With cardiovascular disease remaining the leading cause of death globally, the medical center built AI-powered cardiac risk calculators, redefining preventative cardiology. These tools analyze personal details and compare it to a network of similar patients with known cardiovascular outcomes. This allows for a more nuanced and personalized prediction of 10-year and lifetime heart disease threat, informing lifestyle changes and proactive treatments.
Mount Sinai’s COVID-19 Prediction Model
During the COVID-19 pandemic, Mount Sinai developed machine learning models using the electronic health records of thousands of customers. This software analyzed various factors like history, vital signs, and lab results at patient admission to predict the likelihood of critical events (like intubation) and mortality within clinically relevant timeframes, aiding in case management and resource allocation.
Blue Cross Blue Shield’s Transformation Story
Blue Cross NC employs an award-winning ML platform to proactively identify members at risk of serious health events. It analyzes patterns in their datasets, such as missed follow-up visits or multiple co-occurring conditions. This capability enables their care management teams to intervene earlier. Their “Hospital to Home” program resulted in a 39% drop in 30-day inpatient readmissions for commercial members.
Happily Ever After: The Future Vision
The examples we’ve explored aren’t just success stories. They’re early indicators of a fundamental shift in industry decision-making. As the technology matures, we’re moving toward a new reality where AI predictive analytics and healthcare form a continuous feedback loop that learns and improves with each interaction. Medical businesses effectively implementing these capabilities are seeing considerable advancements in key patient outcomes while simultaneously eliminating operational inefficiencies throughout their systems.
The most significant evolution won’t be technological but cultural. Healthcare facilities will transition from isolated projects to integrated intelligence ecosystems. Moreover, evidence-driven foresight will become embedded in everyday workflows and clinical conversations.
While the destination is clear, many facilities need an experienced partner to guide their changeover journey. Master of Code Global is honored to offer our hand as you take your first steps toward predictive excellence.
The Master Craftsmen: Forging Your Analytics Kingdom
Unlike generic technology implementers, our company approaches healthcare AI consulting through the lens of specific organizational pain points—whether that’s lowering readmission rates for distinct populations, optimizing resource distribution across multiple facilities, or customizing treatment plans according to profiles.
What distinguishes our AI predictive analytics in healthcare practice is our suite of capabilities spanning multiple AI technologies. From sophisticated LLM-based solutions like virtual care coordinators and diagnosis support systems to voice assistants enabling hands-free EHR navigation, we build tools that address the entire ecosystem rather than isolated components.
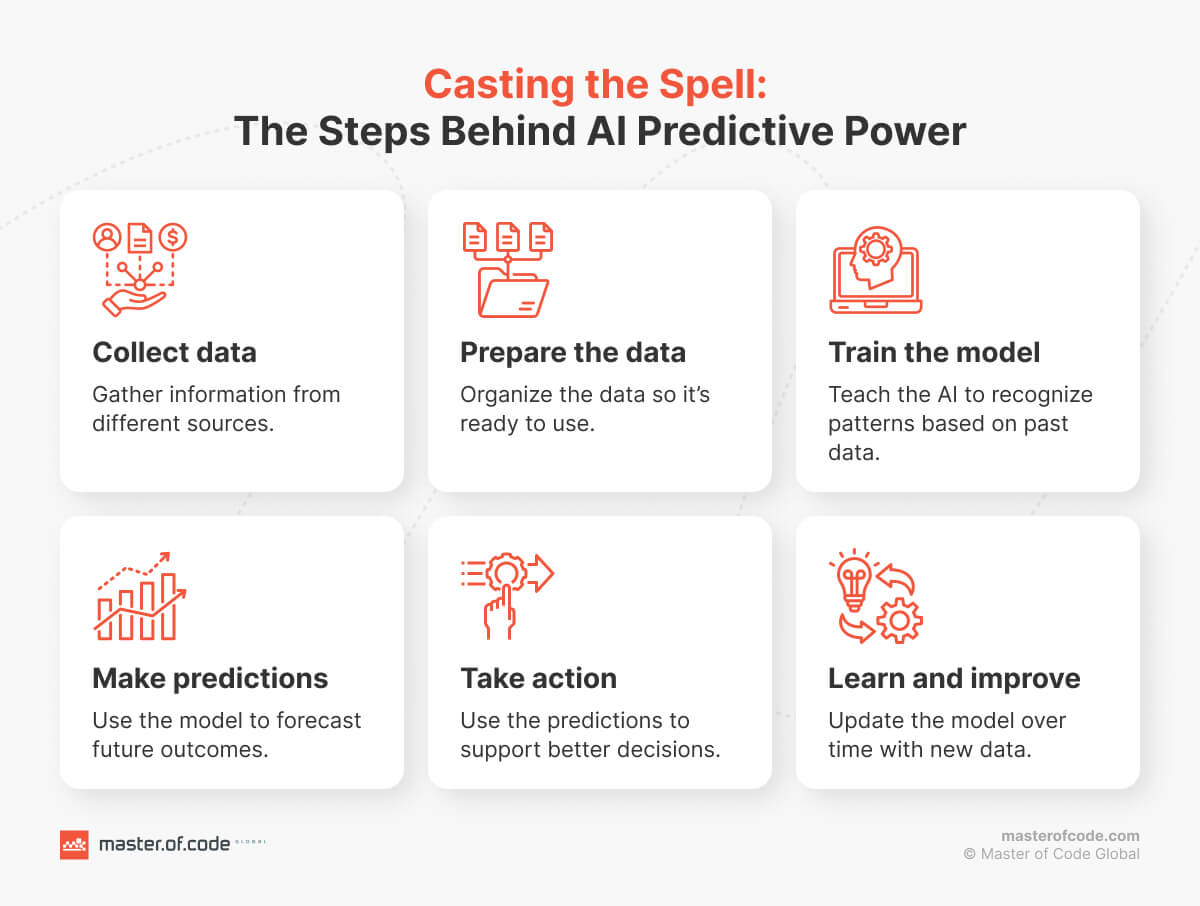
Furthermore, our development process emphasizes high-quality data integration, seamless workflow coordination, and rigorous protection protocols to ensure PHI compliance. Each solution undergoes comprehensive testing. This includes input validation, output filtering, and regular security assessments to protect sensitive information.
Successful AI implementation requires both technical expertise and financial pragmatism. Understanding the cost of implementing AI in healthcare isn’t just about project budgeting. It’s about creating a roadmap that delivers escalating value over time. Our implementation methodology prioritizes high-impact use cases that generate measurable gains. Thus, we ensure that your investment creates both immediate impacts and long-term advantages.
Ready to transform your data from a passive record into an active strategic asset? Contact our experts to discuss how predictive intelligence can help you overcome your most pressing challenges and create a sustainable competitive advantage.
FAQs from Across the Realm
What is AI predictive analytics in healthcare?
It presupposes using AI algorithms to analyze healthcare data (e.g., patient records, medical images) to forecast future health outcomes, risks, and trends. This allows for proactive interventions and more informed decision-making across the entire ecosystem.
What are the benefits of AI-driven predictive analytics in healthcare?
Some of them are more accurate disease detection, personalized treatment plans, optimized allocation of resources, lower costs, and better patient health management. Ultimately, these advantages contribute to a more efficient and customer-centric medical system.
What are the key steps involved in developing forecasting models in healthcare AI?
Stages comprise information collection and preparation, feature engineering, model selection and training, its evaluation, and deployment/integration. Each of these stages requires careful consideration of materials quality, ethical implications, and clinical relevance to ensure reliable and impactful solutions.
What is an example of predictive analysis in the medical domain?
An instance of this is predicting the risk of hospital readmission after discharge based on personal medical history and other factors. This enables targeted support and interventions to prevent costly and detrimental readmissions as well as enhance patient safety.
Future of AI predictive analytics in healthcare?
Emerging opportunities are more precise personalized medicine, earlier disease prevention, proactive and remote monitoring, and optimized system efficiency. The continued evolution of AI promises to unlock even deeper insights and redefine how care is delivered and experienced.
Ready to build your own Conversational AI solution? Let’s chat!