Running a medical business today is a balancing act: surging patient needs on one side, tight budgets and operational hurdles on the other. Artificial Intelligence offers a powerful lever, promising not just to lighten the load but to reshape what’s possible in patient care and operational command. Yet, for leaders like you, the crucial questions remain: “What is the real cost of AI in healthcare, and will that investment genuinely pay off?”
Table of Contents
Master of Code Global’s Proofs of Value: Our Healthcare Case Studies
Forget the hypotheticals for a moment. Let’s look at real-world software we’ve built, where investment turned into an undeniable advantage.
CSource AI Chatbot: Fast, Trusted Answers for the Cancer Journey
The Challenge:
When facing cancer, patients and their families are often swamped by a sea of online information, making it hard to find clear, reliable guidance. CSource wanted to cut through this noise with a central, trusted resource, but their first attempt was more confusing than helpful.
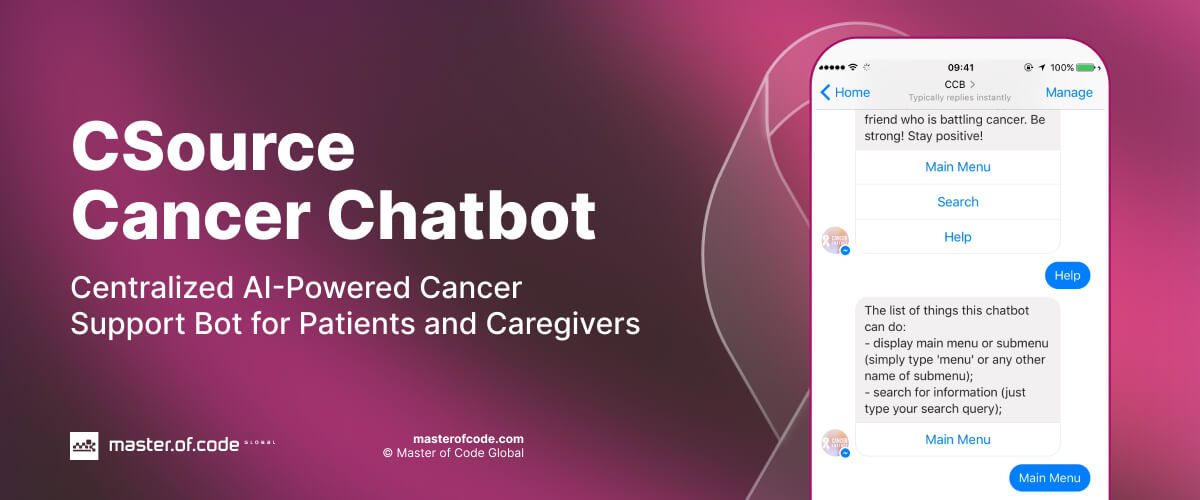
Our AI Solution:
We rebuilt the CSource Cancer Chatbot from the ground up. The new virtual assistant guides users smoothly to vetted information from top organizations like the American Cancer Society. It uses smart, AI-enhanced pathways so people quickly find what’s relevant to their specific situation – whether they’re a patient, caregiver, or supporter – without getting lost or overwhelmed. Also, it was crucial to assure this is a fully HIPAA compliant chatbot.
The Real Value Delivered:
Now, 1,500 people actively use the tool each month, finding clear answers and support. They access 75 core references covering 89 cancer types, meaning less time spent anxiously searching online and more time focused on care. Users navigate easily, never hitting a dead end, which translates to reduced stress and faster access to vital help. This kind of targeted impact helps justify the cost of AI in healthcare when measured against patient outcomes and support delivery.
Project Snapshot (General Market Insights):
Various healthcare chatbot examples like CSource, designed for specialized guidance, typically see development within 3–9 months. Market investment for tools with this level of NLP, curated knowledge, and smart dialogue can range from $40,000 to $150,000, reflecting the tailored expertise built in.
The Pocket-Sized AI Pharmacy: Making Medication Simpler & Safer for an Insurance Leader
The Challenge:
A major Canadian insurer aimed to give their clients a truly smart way to manage medications – something to reduce errors, simplify complex schedules, and provide instant, reliable drug information, all while keeping sensitive data locked down.
Our AI Solution:
We developed a sophisticated iOS app, essentially an AI-driven medicine cabinet. This app doesn’t just remind; it thinks. It proactively checks for risky drug interactions based on an individual’s health profile, helps create personalized medication plans, and even predicts when refills are needed so no one runs out unexpectedly. A built-in virtual assistant provides instant answers to medication questions. This type of solution showcases practical use cases of Generative AI in the pharmaceutical industry when the chatbot component involves advanced conversational abilities.
The Real Value Delivered:
The impact was clear: calls to the insurer’s support lines about medication questions dropped by 43%, because people got their answers directly and easily from the app. Over 1 million medication records are managed securely (with zero data breaches), building deep user trust. And, thanks to AI, the drug database expanded by 320%, meaning users always have more comprehensive, up-to-date information for safer medication use.
Project Snapshot (General Market Insights):
AI-driven platforms for complex tasks like personalized medication management, with predictive analytics, secure data handling, and regulatory alignment, generally involve 6–12+ month timelines. Prices in the market for these sophisticated patient assistant applications often start around $70,000 and can exceed $250,000, reflecting the depth of AI required to deliver truly personalized and proactive care. But the final estimation of the cost of implementing AI in healthcare depends on dozens of factors, so it’s impossible to forecast the price without analysing the project scope.
These weren’t just software rollouts. They are strategic shifts where intelligent technology delivers distinct improvements to people’s lives and an organization’s performance. Now, let’s break down the typical components that make up the cost of artificial intelligence in healthcare. Explore how to prove that investment generates the kind of clear value you’ve just seen.
What Really Shapes the AI Pricing?
So, you’ve seen what technology can do. The next logical question is, “What does it take to get there?” The truth is, artificial intelligence isn’t an off-the-shelf product with a fixed price tag. The cost of AI in healthcare is a spectrum, shaped by your specific ambitions, the intricacy of the solution, and the digital groundwork you already have in place. Think of it like building a specialized medical facility. The needs for a local clinic versus a cutting-edge research hospital will dictate very different blueprints and budgets. Let’s lift the hood and look at the core components that determine your investment.
1. Data: The Lifeblood of AI
- Acquisition & Preparation: Well-performing software is non-negotiable; it absolutely relies on high-quality data. This means dedicated effort upfront to gather, clean, label, and, critically, anonymize your data to make it fit for purpose. If you need to acquire specialized datasets, that’s another factor. Skipping thorough preparation is like building that state-of-the-art hospital on shaky ground – the outcomes will be compromised. You need robust, documented processes here.
- Storage & Management: Healthcare data demands Fort Knox-level safety and compliance. This translates to secure, excellently-managed storage solutions, whether on-premise or in the cloud. It’s not just about space. It’s about accessibility, integrity, and unwavering adherence to regulations like HIPAA or GDPR. This isn’t an area to cut corners and save on the cost of implementing AI in healthcare, as patient trust and legal standing depend on it.
- Integration: Often, the most powerful AI insights emerge when you connect diverse data streams – electronic health records (EHRs), lab information systems (LIS), medical imaging archives (PACS), pharmacy data, even information from patient wearables. Weaving these disparate sources together into a cohesive, secure data fabric is a significant technical undertaking. But it’s frequently where the real value of tech kicks in.
2. Talent: The AI Brains Trust
- Specialized Experts: Building an effective system requires more than just good coders. You’re looking at data scientists who can unearth patterns, machine learning (ML) engineers who can build and train robust models, and increasingly, AI ethicists to ensure fairness and transparency. Securing this A-team, whether developing in-house or through a specialized partner, is a big but vital investment.
- Domain Champions (Your Clinical & Admin Experts): A model built in a vacuum rarely succeeds in healthcare. The active involvement of your clinicians, nurses, and administrators – the people who will use the AI or be impacted by it – is absolutely crucial. Their real-world insights guarantee the solution is relevant, practical, and genuinely solves the right problems.
3. Technology & Infrastructure
- AI Platforms & Tools: You’ll need to choose the right “horsepower” for your solution. This could involve leveraging powerful cloud services (like those from AWS, Azure, or Google Cloud) with their pay-as-you-go models and scalable resources, or, for specific needs, investing in on-premise hardware and custom-built platforms. These foundational choices have a direct influence on the cost of AI in healthcare, depending on scale, security needs, and flexibility.
- Development & Customization: While some off-the-shelf components exist, healthcare solutions almost always require significant customization. Your unique workflows, patient populations, and strategic goals mean that tailoring the model is not just common, it’s essential for it to deliver real value.
4. Algorithm Development & Model Training
- Model Complexity: The sophistication of your system directly influences its portion of the cost of AI in healthcare. A relatively simple tool to triage administrative tasks will have a different development path and pricing structure than a complex deep learning model designed to detect subtle anomalies in medical images or predict sepsis risk hours in advance.
- Training Time & Computational Power: Efficient and accurate models don’t become smart overnight. They need to “learn” from vast amounts of data, which requires significant processing power (often specialized GPUs) and time. The more complex the model and the larger the dataset, the more intensive this training phase becomes.
5. Integration & Deployment
- Workflow Integration: This is a critical make-or-break point for many projects. The most brilliant AI is useless if it’s clunky or disruptive for your staff. Such tools must fit seamlessly and intuitively into existing clinical workflows. This often requires careful design, user testing, and iteration. This is especially true for AI in telemedicine, where user experience for both patient and provider is paramount.
- System Interoperability: Your new solution can’t be an island. It needs to “talk” securely and efficiently with your core systems – EHR/EMR, billing platforms, lab software, etc. Ensuring this smooth data exchange is vital for a connected healthcare experience.
6. Compliance, Security & Ethics
- Regulatory Adherence (HIPAA, GDPR, etc.): You need to implement and rigorously maintain robust compliance and security mechanisms. This includes strong authentication, safe data connections, end-to-end encryption, audit trails, bias detection in algorithms, and processes that ensure decision-making is as transparent as possible. This isn’t a one-time checklist; it’s an ongoing commitment. For specific applications like advanced AI integration for healthcare fraud, these mechanisms are even more critical.
- Ongoing Audits & Updates: The regulatory landscape is always evolving, and smart models can drift or reveal unintended biases over time. Regular audits, updates, and ethical reviews are necessary to keep your solutions safe, fair, and compliant.
7. Maintenance, Monitoring & Evolution
- Keeping the AI Sharp: Any models are not static. Their performance can degrade over time as new data patterns emerge (a concept known as “model drift”). They require ongoing monitoring, periodic retraining with fresh data, and updates to maintain their accuracy and relevance.
- Support & Iteration: All applications of Generative AI in healthcare are rarely a “set it and forget it” deployment. It’s a journey. You’ll need ongoing assistance and a plan for iterating on the solution as you learn more and as your needs evolve. Continued investment in evolution and support is often an overlooked part of the cost of AI in healthcare.
8. The “Extra” Expenses (Often Overlooked, Always Crucial)
- Change Management & Staff Training: Introducing new tools means new ways of working. Investing in thorough training for your team and a thoughtful change management strategy is essential for adoption and to ensure everyone can effectively use these powerful new capabilities.
- Dedicated Management & Governance: Ambitious medical projects are complex, with many moving pieces. Strong, dedicated oversight is what takes an AI concept from a bright idea to a real-world success. This means having skilled hands steering the workflow: meticulously planning, keeping all teams aligned, controlling budgets and timelines, and making sure clear decisions are made to deliver the results you expect.
Healthcare Market Ballpark Figures (What You Might Expect to Invest)
Understanding the pillars above helps frame why the cost of artificial intelligence in healthcare can vary so widely. Generally, on the market, you might see:
- Minimum Viable Products (MVPs): To test an idea or a core feature, initial projects often land in the $20,000 to $100,000 range. These help validate assumptions before larger commitments.
- Moderately Complex Solutions: For intelligent tools focused on specific departmental needs, more sophisticated chatbots, or initial predictive AI in healthcare models, investments typically range from $80,000 to $350,000. These products offer more robust functionality and integration.
- Highly Complex, Enterprise-Wide Systems: For more comprehensive platforms, the investment level is naturally higher. This category includes systems designed for large-scale population health analytics, or advanced AI diagnostic tools deeply integrated into daily clinical workflows. It also covers bespoke solutions that require significant custom algorithm development from the ground up. For such projects, initial investments readily start at $300,000 and can easily extend beyond $1,000,000—sometimes considerably more—particularly when the scope involves a very large scale of groundbreaking innovation.
A Necessary Note: These are broad market estimates to provide context. The precise investment for your AI initiative will always depend on your specific requirements, the complexity of the problem you’re solving, and the existing technological landscape within your organization.
The ROI of Intelligence in Healthcare – Why the Investment Pays Dividends (Often Exponentially)
Meet Eva. She’s the Chief Operating Officer at a busy regional hospital network. Every day, she navigates a complex maze of budget constraints, staff scheduling puzzles, and the constant drive to deliver exceptional patient care. She sees her talented clinicians stretched thin with administrative tasks, worries about optimizing patient flow through the ER, and dreams of proactively managing community health rather than just reacting to illness. Eva has been exploring technology trends, asking: “Beyond the initial spend, what’s the real, tangible return for my hospitals, my staff, and my patients?” Like many leaders, she’s weighing the cost of AI in healthcare against its potential to deliver measurable improvements in care quality and operational efficiency.
Like Eva, you might initially focus on budget savings – and intelligent automation certainly delivers there. But its true value ripples out far wider, creating compounding returns that can redefine your organization’s capabilities and impact.
Financial Returns: More Than Just Shaving Costs
- Operational Boosts for Your Team: AI handles routine work like data entry and initial administrative screening. This directly frees your expert medical staff to concentrate on complex patient care and critical decision-making—the work only they can do. Using AI in healthcare administration can significantly amplify these boosts.
- Catching Costly Errors, Earlier: Intelligent systems can act as a vigilant co-pilot, flagging potential drug interactions or subtle diagnostic indicators. This helps prevent medical mistakes, leading to fewer expensive-to-be-fixed complications and better patient safety.
- Smarter Resource Use, Day In, Day Out: AI predicts patient surges, optimizes staff schedules, and manages inventory more precisely. This means your existing resources—beds, equipment, and personnel—are used more effectively, stretching budgets further.
Clinical & Patient Triumphs: Where AI Touches Lives
- Diagnosing Faster and More Accurately: A model analyzes medical images and patient data to spot early signs of disease, often faster than traditional methods. Earlier, more accurate diagnoses significantly improve patient outlooks and treatment options.
- Plans Tailored to the Individual: AI supports the shift to personalized medicine. By assessing individual patient data, including genetics and lifestyle, it helps craft tactics optimized for each person.
- Empowering Patients in Their Health Journey: Smart tools give patients direct access to reliable health information and support for managing their conditions. This active involvement often leads to better treatment adherence and higher satisfaction.
- Proactive Care to Prevent Serious Illness: AI can identify individuals at high risk for conditions like diabetes or heart disease before major problems arise. This enables timely, targeted interventions, keeping people healthier and reducing the long-term burden of chronic illness.
Strategic Advantages: Building a Future-Ready Organization
- Gaining a Competitive Edge: Implementing AI demonstrates a commitment to cutting-edge care. This attracts top medical professionals and patients seeking the most advanced treatment options available.
- Fueling Innovation and Discovery: AI accelerates research by rapidly analyzing vast, complex datasets. Your organization can contribute more effectively to medical breakthroughs and bring new treatments to patients sooner.
- Improving Staff Satisfaction and Retention: By automating mundane tasks, artificial intelligence allows clinicians to focus on more meaningful patient interaction and complex care. This helps reduce burnout, a critical factor in retaining valuable medical staff.
Think of it this way: investing in AI is like your organization upgrading from a fleet of horse-drawn carriages to deploying the first self-driving electric vehicles. The initial investment in the new technology, infrastructure, and training is undoubtedly more significant. But the leap in speed, precision, safety, efficiency, and the entirely new possibilities for how you operate and serve your community are simply incomparable. The “cost” is an entry point to a new era of capability.
Healthcare With and Without Intelligent Augmentation
The path forward in the medical industry is increasingly diverging, creating two distinct operational realities: one reliant on traditional methods, the other amplified by intelligent augmentation. And this is no longer just about adopting new software. Virtual assistants change how care is delivered, data is incorporated, and innovation is pursued. The gap between these two approaches is widening, and understanding this “AI divide” is crucial for any leader planning for a resilient future. Here’s a closer look at these contrasting landscapes.
Feature Area | Healthcare WITHOUT AI | Healthcare WITH AI |
---|---|---|
Data Utilization | Data often sits in silos, difficult to access or analyze comprehensively. This leads to fragmented patient views and slow, reactive decision-making. | Data is integrated and readily accessible, providing holistic patient views and generating actionable insights in real-time for informed decisions. |
Care Philosophy | Primarily reactive: care focuses on treating symptoms and conditions after they become apparent, often resulting in delayed interventions and more complex cases. | Proactive & predictive: AI identifies health risks and predicts potential issues before they escalate, enabling early interventions and preventative care strategies. |
Operational Processes | Heavy reliance on manual processes for administrative and clinical tasks. This is time-consuming, prone to human error, creates bottlenecks, and contributes to staff burnout. | Automates routine and repetitive tasks, streamlining workflows. This reduces errors, enhances efficiency, and frees up human staff for complex, high-value activities. |
Treatment Approach | Tends towards generalized treatment protocols; “one-size-fits-many” approaches with limited capacity for deep, individual patient customization. | Facilitates hyper-personalized medicine; treatments are tailored based on individual genetic makeup, lifestyle, specific conditions, and predictive responses for optimal outcomes. |
Clinician Experience | Clinicians spend a significant portion of their valuable time on administrative burdens like paperwork and data entry, reducing time available for direct patient interaction and care. | Administrative tasks are significantly reduced and streamlined. Clinicians can dedicate more focus and time to direct patient care, complex diagnoses, and empathetic engagement. |
Innovation & Research | The adoption of new medical research and translation of discoveries into clinical practice is often a slow, laborious process, delaying patient access to the latest advancements. | Accelerates the journey of research from lab to bedside. AI helps analyze complex data faster, enabling quicker adoption of innovations and improving patient access to cutting-edge care. |
Overall Outlook | It’s like navigating complex medical situations with a flickering candle – visibility is limited, progress is painstaking, and critical details can be easily missed. | This is more like navigating already with a suite of AI-powered diagnostic tools and predictive analytics – clarity is improved, speed is increased, and precision in care delivery is paramount. |
AI in the Enterprise Healthcare Ecosystem
While AI can solve specific problems within a department, its true strength in a large healthcare enterprise is its ability to drive systemic change. When scaled, it doesn’t just offer isolated fixes; the model can reshape how your entire organization works, makes decisions, and cares for large populations. Here’s how intelligent automation delivers powerful capabilities across a multi-facility healthcare enterprise.
- Managing the Health of Entire Communities: AI systems can scan and analyze vast amounts of health information from across your region – patient records, public health statistics, even local environmental data. This allows your enterprise to see emerging patterns, like early signs of a flu outbreak spreading across several towns you serve. When viewed at scale, the cost of AI in healthcare can be more easily justified by its ability to predict and manage population-level health events with precision.
This helps your hospitals prepare weeks in advance, perhaps by adjusting ER staffing across multiple sites or ensuring enough flu tests are available. It also allows you to direct community health programs to specific neighborhoods showing high rates of chronic conditions like diabetes, helping people manage their health better and potentially reducing future hospitalizations across your network. - Running Smoother Operations Across All Your Facilities: Imagine AI looking at the real-time needs and resources across all your connected hospitals and clinics. It can identify that a specialized mobile imaging unit is underused at one site but urgently needed at another, or that one hospital’s emergency department is overwhelmed while a nearby facility has capacity. Smart tools can also track how medications are being used enterprise-wide. This kind of optimization directly influences the overall cost of implementing AI in healthcare, helping organizations avoid waste while boosting responsiveness.
This means expensive equipment gets used more efficiently instead of sitting idle, and patient loads can be better balanced. Your whole system makes smarter decisions about purchasing and distributing medicines, so less is wasted and crucial drugs are consistently available where your patients need them. - Making Smarter Decisions with Unified Enterprise Data: AI thrives on good data, and at an enterprise scale, it can help you build systems where information from all your different parts—hospitals, labs, outpatient clinics, administrative departments—is brought together securely and makes sense. Information that was previously stuck in one department’s computer system can now paint a bigger picture.
For example, your financial team can see the true cost implications of a new surgical technique being trialed in several hospitals, or your central quality improvement group can compare patient recovery times for a specific procedure across all your surgical units. This allows leaders throughout your enterprise to make better-coordinated decisions based on a fuller understanding of what’s happening system-wide. - Ensuring Consistent, High-Quality Care Everywhere: Across a large enterprise with many doctors and nurses, it can be challenging to ensure every patient benefits from the very latest proven medical guidelines. AI can be built into your digital clinical systems to gently prompt or alert staff if a planned action deviates from an established best-practice protocol – for example, for sepsis management or post-operative care.
This helps ensure that every patient, no matter which of your facilities they visit or which clinician they see, receives care that aligns with the highest, most current medical standards. This consistency can directly lead to fewer complications and better recovery for more patients. - Driving Your Own Medical Advances: AI tools can give your research teams the ability to analyze large volumes of anonymized patient data collected across your entire enterprise. By examining this rich data, your researchers might uncover that an existing medication has unexpected benefits for a certain patient subgroup, or identify specific local factors that dramatically speed up recovery from a common surgery.
This makes your own healthcare enterprise a hub for discovering new, effective ways to care for the specific populations you serve. These unique insights, developed within your system, can directly improve how you treat people and establish your organization as a pioneer.
Your Path to AI-Powered Healthcare: From Planning to Reality
Bringing intelligence into your medical system, especially across multiple facilities, isn’t an overnight switch. It’s a clear process: start with solutions that solve real problems and show quick, solid results, then build on those wins to expand its reach. Dealing with complex data, fitting new tech into old systems, and getting everyone on board – all while managing the cost of implementing AI in healthcare – means having skilled tech partners by your side. It isn’t just helpful, it’s often what makes these big projects actually work well and deliver better care.
Innovative tech is changing the sector, no question. The real question is how your organization will use it. This is about investing in a stronger future for your operations, better outcomes for your patients, and more support for your staff. Curious about what this kind of AI investment could do for you? Let’s talk, straight up. Our healthcare AI consulting services were designed to address just that. We can look at your specific needs and show you how we help turn good ideas into working models, as well as fully understand the real cost of AI in healthcare so you can plan with clarity and confidence. Get in touch for an initial strategy discussion by submitting the form below.
Frequently Asked Questions
How does AI reduce costs in healthcare?
Think about all the administrative tasks, like paperwork and scheduling – AI can automate a lot of that, freeing up your staff’s time and reducing labor expenses. It also gets smart about using your resources; for example, ensuring operating rooms are used efficiently or that staff schedules match patient demand. This way, you’re not paying for unused time or space. When AI helps doctors spot diseases earlier or catch potential medication mistakes, it means patients are less likely to face expensive complications or need long hospital stays.
Technology can also manage your medical supplies better, predict when expensive equipment needs a check-up to avoid big repair bills, and support your efforts to keep people healthy with preventative care, which usually costs much less than treating serious illnesses. For instance, if an intelligent tool assists in getting medical coding right the first time, you’ll see fewer denied insurance claims and faster payments.
How much does a healthcare chatbot cost?
The price tag for such a bot really depends on what you want it to do for your patients or staff. If you need a simple tool that just answers common questions using basic language understanding, you might be looking at an investment between $15,000 and $40,000. But for a more advanced assistant – like the CSource Cancer Awareness tool we built – this tag may be much higher.
As the solution can have detailed conversations, securely connect with patient records for personalized information, help people understand symptoms, and meet all the important privacy rules like HIPAA, the AI chatbot cost can range from $40,000 to $150,000, or sometimes more. What makes the price go up or down? Things like how much information the chatbot needs to know, whether it needs to communicate in different languages, and just how “smart” its AI needs to be to really understand and help people effectively.
What are the cost implications of implementing AI in healthcare?
When you choose tailored AI solutions for your healthcare services, you’ll typically partner with a development team. Your initial investment then covers several key areas. This includes the collaborative design and building of software tailored precisely to your needs. It also involves preparing your patient data and setting up secure systems. A crucial part is integrating these new custom intelligent tools smoothly with your existing hospital platforms, like EHRs. After this setup, you will have ongoing expenses. These are for maintaining and updating the AI solution.
You’ll also need to train your staff and ensure you always meet healthcare regulations, such as HIPAA. While these are important financial points to consider, developing custom AI with a trusted partner often brings significant positive returns. You can look forward to smoother operations, better patient care, fewer costly errors, and even new service opportunities.
Ready to build your own Conversational AI solution? Let’s chat!